Lauren Maffeo on Data Governance from the Ground Up
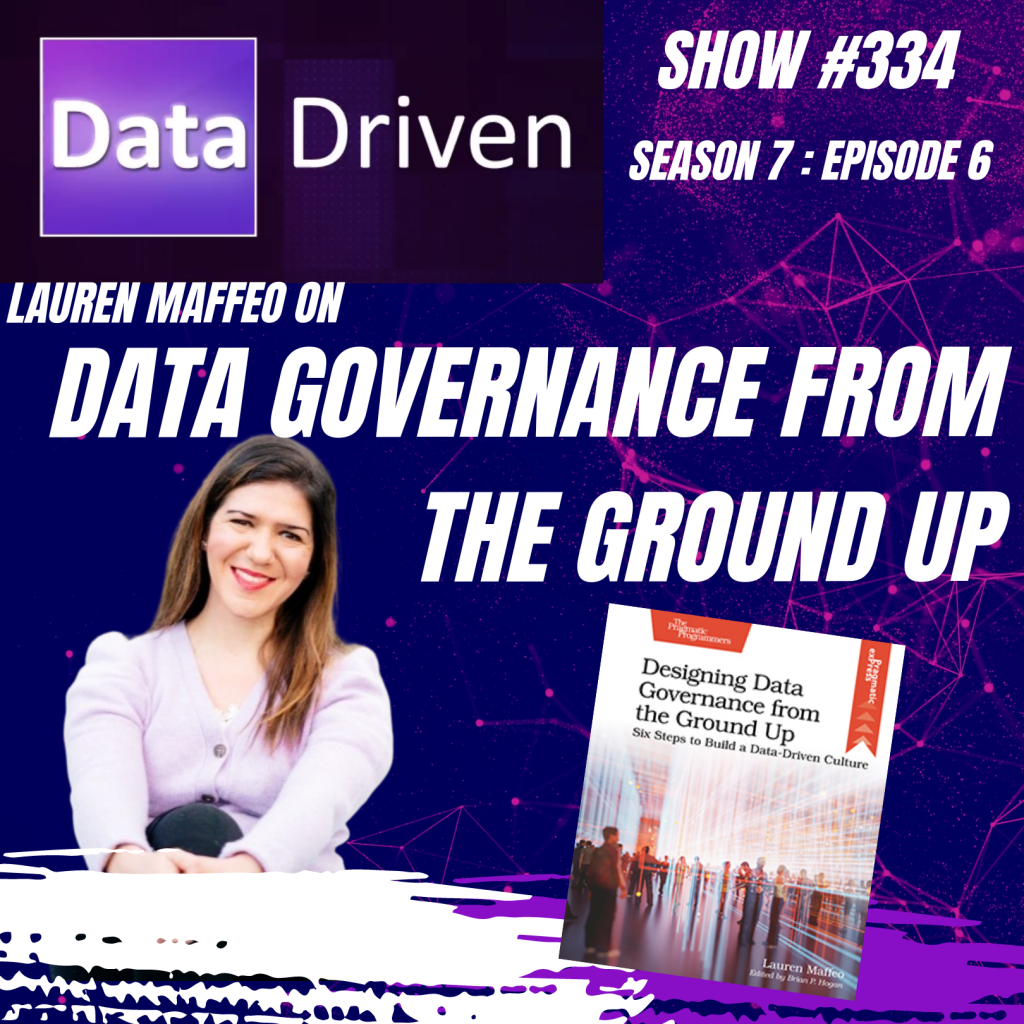
In this episode of Data Driven, Frank and Andy Leonard are joined by guest speaker Lauren Maffeo to discuss data governance from the ground up. The conversation revolves around the importance of data governance in relation to generative AI, copyright infringement, and protecting consumer rights.
They explore topics such as the need for proactive cybersecurity measures, the challenges faced by startups in implementing data governance, and the cultural transformation required for successful implementation.
Overall, it is a thought-provoking discussion that provides insights into the complexities and potential solutions related to data governance in today’s data-driven world.
Moments
00:05:49 Civic Tech serves the public through technology.
00:07:50 Data governance: a holistic, cultural business strategy.
00:12:25 Data as tangible asset, managing as product.
00:14:38 Implementing data governance: start small, connect to business.
00:20:34 Data growth, lack of management, legislative progress. Clear framework for data quality needed.
00:25:14 Startups prioritize innovation for survival. Large industries restrict innovation due to regulation. Motivations and context are key in governance.
00:28:54 Data governance and copyright infringement in generative AI. The future of consumer rights and cybersecurity.
00:33:44 Encourage caution with sharing proprietary information
00:36:36 Bias in AI and data governance intertwined. Risk reduction, troubleshooting. Not all intent is negative. Challenges in data work solvable. Nonprofits and cybersecurity models for governance.
00:40:38 Encouraging shift in conversation about data governance.
00:44:34 Data found me, sparked interest in AI.
00:49:20 Technology saves time, allowing for more productivity.
00:54:03 Adopting foster pets: fun without long-term responsibility.
00:55:57 Connect on LinkedIn, visit Pragprov.com, feedback welcome.
Transcript
On this episode of data driven Frank and Andy interview Lauren
Speaker:Mafayo author of Designing Data Governance from the Ground
Speaker:Up Data governance has become more pressing of late,
Speaker:what with all the advancements in generative AI systems.
Speaker:Tune in for a fascinating look at data governances, civic
Speaker:technology, and more.
Speaker:You. Hello, and welcome to Data Driven
Speaker:Podcast. We cover the emergent fields of data science,
Speaker:AI, and machine learning. Today,
Speaker:I'm here with Andy. My voice is a little crackly because of a
Speaker:sinus infection, but it's all
Speaker:good. I've gotten on the meds and I am definitely feeling like
Speaker:I'm on the mend. How are you doing, Andy? I'm well,
Speaker:Frank. And I just heard how you were doing. Actually, I knew a little bit
Speaker:about it because you texted me when you were in the throes of it, and
Speaker:I knew something was up because usually you communicate
Speaker:more. I was like, Frank's down for the weekend. And
Speaker:I know you've been having very busy weekends the past
Speaker:little bit for something that people will know more about
Speaker:later, right? Much later, probably. But it's all
Speaker:good. It is all good so far. It's ended well. So for
Speaker:folks that we're going to release this episode, we're recording this on
Speaker:July 17, we're going to release this probably on July
Speaker:18. And you'll hear me refer to a legal
Speaker:case. It looks like that will be resolved this
Speaker:week, hopefully in one form or the other, and it's gone our way. That's all
Speaker:I can say right now. But it is good news. Speaking of
Speaker:good news, we have with us an excellent guest who's
Speaker:based in the DC area. So not that far from Chateau
Speaker:Lavinia. It is Lauren.
Speaker:Sorry, she will correct me, but she's a published
Speaker:author. Her book just came out talking about designing data
Speaker:governance, which is a topic that just more and more
Speaker:keeps coming up. And I think that if you're a data engineer and you think
Speaker:I don't have to worry about that hold up. Maybe you should need to worry
Speaker:about that. Even data scientists? Especially data scientists, I would
Speaker:say, and doubly so if you're in the
Speaker:generative AI space. I think we'll see what we get into that.
Speaker:And she has a very interesting background, so I'll let her explain
Speaker:it. Welcome to the show, Lauren. Thank you guys, for having me. I'm really
Speaker:excited to be here and to chat with you all. Yeah, likewise,
Speaker:likewise. So your background is
Speaker:amazing. You studied overseas at Cambridge,
Speaker:I think. At LSE
Speaker:and the London School of. Economics, which is like, wow,
Speaker:I half expected you to have a British accent, honestly, because I wasn't
Speaker:sure. And you also have spent
Speaker:some time doing arts and design, so
Speaker:I found that fascinating too. I actually
Speaker:am a service designer in my day job, and so I work very
Speaker:closely with data scientists and engineers to
Speaker:design things like pipelines, cloud architecture,
Speaker:environments, different service models for
Speaker:chief Data Officers. And so I always say as a service
Speaker:designer that I'm the user advocate on a project. I'm the person
Speaker:who is tasked with helping the client define who their key
Speaker:user groups are. And once I do that, I conduct user
Speaker:interviews with people who fit those demographics to figure out what
Speaker:they like or dislike about a product or service. I capture
Speaker:the results of those interviews and design assets like personas and journey
Speaker:maps. And then ultimately I do work with people like
Speaker:you, data architects, engineers, scientists
Speaker:to build a product that will hopefully solve the pain points that
Speaker:we uncovered in the user research. Fascinating.
Speaker:And you were in the Civic Tech space if memory serves as well, which
Speaker:is a fascinating space that once upon a time
Speaker:I was on the Microsoft Civic Tech team. Yes, I am. So
Speaker:I work for an organization called Steampunk and we're a human centered design
Speaker:firm that builds solutions for federal government
Speaker:agencies because as we all know, the federal government is
Speaker:the most progressive when it comes to tech and so they
Speaker:barely need us at all. But the reality actually is that they
Speaker:need us quite a bit and that we very often come in and
Speaker:have that human centered approach that many of their tools
Speaker:were just not built with. And so then we come in and often
Speaker:try to improve them and improve the user experience.
Speaker:And user experience in that context is really about
Speaker:getting the right services to the American public, which I
Speaker:think is what makes the work so interesting. It's not commercial products, it's
Speaker:things like improving unemployment benefits and how
Speaker:easily it is for people to, how easy it is for people to access them,
Speaker:improving the ease with which you can send folks overseas in official
Speaker:roles, defining the service offerings
Speaker:that a Chief Data officer is going to provide its
Speaker:colleagues. And so the problems that you solve in Civic Tech I think
Speaker:are really fascinating. And I think COVID was the
Speaker:final confirmation that all of these systems are long
Speaker:overdue for major upgrades which we are seeing
Speaker:the influx of now. Yeah, you don't have kind of good
Speaker:user design or good user experience as part of the RFP
Speaker:that went out for building these large federal systems. That made was
Speaker:probably not a bullet point on the list, not at
Speaker:worse. So for those not familiar with Civic
Speaker:Tech, how would you define it? I would define
Speaker:Civic Tech as technology which exists to serve
Speaker:the public. And the public is very broad. I would define the
Speaker:public further by saying it's citizens of any
Speaker:country or area where
Speaker:the tech exists. And so for instance, Civic Tech
Speaker:encompasses the tech in a town
Speaker:that my hometown, for instance, NATIC, Massachusetts might use to
Speaker:serve residents of NATIC. So this could be anything from
Speaker:tech that allows people to pay their bills online
Speaker:to applying for benefits. And then likewise I
Speaker:work as a designer in the federal space. And so I work with US
Speaker:federal agencies to improve the
Speaker:way that they deliver services to the American public. And the
Speaker:public in this case, is any American who needs to use
Speaker:those services. But then we get more granular about who those
Speaker:particular user groups are. So, for instance, I have worked on
Speaker:many projects in the past with the Department of Agriculture, and within
Speaker:the Department of Agriculture there are many different
Speaker:subdivisions that serve different user groups. And
Speaker:so then I will work with my client to define what those user
Speaker:groups are and figure out how we can tailor a user
Speaker:experience and a product to meet those unique needs. But I would
Speaker:broadly define civic tech as any technology which
Speaker:serves the public. And the public can then be further
Speaker:defined into groups based on things like geography, but
Speaker:also things like role, the day to day experience,
Speaker:things like that. That's a good definition because it
Speaker:used to be very nebulous in terms of what it meant and the implications
Speaker:thereof. But I like your definition. It's probably the most cogent
Speaker:I've heard to date of the field. Thank you.
Speaker:Now this explains so how did you get into data governance, right? Because
Speaker:this is something well, let's start before we do that. How would you
Speaker:define data governance? I love the fact that you
Speaker:start the conversation by asking me to define it, because I think like
Speaker:many terms in tech, it is often left undefined. And that's
Speaker:why there's not only a lot of confusion about it, but also a lot of
Speaker:resistance to it. I think people have in their heads that governance is
Speaker:purely compliance and that it is a blocker
Speaker:to innovation and to tinkering. Other people think
Speaker:that it is something that you can quote unquote, ship after
Speaker:deployment. And I have had C suite leaders say as much. They've
Speaker:said things like, we'll do data governance later, or
Speaker:we will deliver it in the next contract after
Speaker:production. And that refrain is still unfortunately
Speaker:common. So I define data governance as the strategy you
Speaker:have to encompass the people, processes and
Speaker:tools that help you manage your data at scale. And I often
Speaker:say manage your big data at scale. Big data, as we
Speaker:know, is another buzzword that often means both everything
Speaker:and nothing. But I use big data in this context because the
Speaker:reality is that most organizations have more data that
Speaker:they both ingest and produce than ever before.
Speaker:It is too big for one person
Speaker:or one team to manage on their own. And that's why you do need this
Speaker:holistic data governance strategy that is really
Speaker:a business strategy before a technical
Speaker:strategy. Your data governance should never be divorced from what you're
Speaker:doing in development and production environments. It should be
Speaker:integrated into those environments. But at the same time,
Speaker:I think people make a mistake when they think of data governance not just
Speaker:as pure compliance, but also purely as a technical problem to
Speaker:solve. Because the more complicated reality is that it's a
Speaker:cultural transformation that your organization needs
Speaker:to be invested in from the top down. And that's really how you
Speaker:gain success from data governance. Now, that's a good way to put it.
Speaker:And that's why I wanted to define it, because it doesn't have a very firm
Speaker:definition, right. My definition, that my operating
Speaker:definition is pretty close to yours. I'll say it's really because
Speaker:in my day job at Red Hat is like they ask, well,
Speaker:what does your product do for data governance? And I kind of laugh and say,
Speaker:well, not really much, because
Speaker:data governance is largely around,
Speaker:yes, it's people, processes and technology. But 80% of that is
Speaker:nothing is not technology. Right.
Speaker:And you need a vehicle to make it happen in
Speaker:the technology space. But the people in process part,
Speaker:those are going to be the hard ones. Absolutely. And that's why
Speaker:it is so tricky. I think it's also why
Speaker:relatively few organizations have made a lot of headway. And that's also
Speaker:why I think it's really important to frame data governance as a
Speaker:cultural transformation that you can design and embed
Speaker:into your business strategy. You really cannot
Speaker:separate the two. I think a lot of people have been saying that
Speaker:for quite some time now, but we're really seeing the
Speaker:results of that and rather the results of not
Speaker:doing that now we are in a pseudo
Speaker:recession, if not an actual recession. Tech organizations have certainly been
Speaker:acting like there's a recession with both layoffs of
Speaker:employees, but also in their buying behaviors
Speaker:and in not buying as many cloud tools and
Speaker:pieces of software that they used to. And so it's more important than ever
Speaker:that whatever technology you're investing in is
Speaker:producing tangible outputs for your organization. And so
Speaker:we're seeing the consequence of trying to divorce data
Speaker:governance from your business strategy. It's just no longer
Speaker:an option to separate the two. No, I totally agree.
Speaker:And Andy looks like he has a question, but I want to get this out
Speaker:there. I think part of it is that a lot of organizations, and I mean
Speaker:legacy organizations probably, I would say federal, it would definitely fall on this,
Speaker:is that it's only been in the recent years,
Speaker:maybe decade, that we've thought of data as an asset
Speaker:as opposed to a byproduct of some other process.
Speaker:And maybe that's it now it's
Speaker:something of value. And as with anything of value, you probably should
Speaker:have processes not guards around it, but gatekeepers or gates
Speaker:around it just to make sure it's not wasted, it's not
Speaker:contaminated, that sort of thing. That's where my head is at.
Speaker:I agree with that. I think data as an actual
Speaker:tangible asset is a relatively new concept, certainly
Speaker:within the last decade. And I think what's also new about it
Speaker:is the pure volume of data that exists in the world
Speaker:today, there is more data produced and
Speaker:ingested than ever before, and that number is
Speaker:certainly not going to go down. When you think about all of the Internet connected
Speaker:devices that exist, when you think about the explosion of remote work and the
Speaker:fact that now employees are doing work for their
Speaker:organizations on private devices, which means that you can be
Speaker:having organizational data that exists in several locations,
Speaker:which is a very tangible reality. And then I
Speaker:think that lends itself to the broader conversation
Speaker:that I see happening in data circles now about managing data more
Speaker:as a product and less as a service, which is an approach
Speaker:that I largely support because a big part of what you need to
Speaker:do to be successful at data governance is
Speaker:defining clear data domains and subdomains within
Speaker:your organization. These are the key areas that your
Speaker:organization collects data on, and then it gives you a way of
Speaker:categorizing them more clearly, rolling them up to
Speaker:specific owners. These would be equivalent to your product managers if we're
Speaker:using the product analogy. So there's a lot being done to
Speaker:reframe big data in this way as an
Speaker:asset that you manage like a product. And I think there's a lot of
Speaker:value to that, rather than the top down data
Speaker:as a service model that begins and ends with it
Speaker:and begins and ends with people who really lack the
Speaker:context to make those decisions about data and
Speaker:its quality across domains, I. Think that's really
Speaker:important. Lauren and what would you say
Speaker:to an enterprise or just maybe a small
Speaker:to medium sized company that says, yeah, we
Speaker:understand all of that and they kind of give mental assent to
Speaker:it, but they think about their culture and the way they've always done
Speaker:things and they can't bridge that
Speaker:gap? That's a great question because I
Speaker:think that is realistically. Where the biggest blockers
Speaker:occur, people are messy, they're
Speaker:intangible, they all have different motivations, even if
Speaker:they work for the same organization, they not only have different roles,
Speaker:but they have different end goals. Very often you have people
Speaker:in organizations who do not want change, they
Speaker:want things to say the same, they have a vested interest in it, even
Speaker:if that is arguably not what is best for the organization in
Speaker:the long run. You will have people who are invested
Speaker:in not changing the status quo, especially as it pertains
Speaker:to data. I think a lot of that comes down to the fact that data
Speaker:governance has not been practiced to the degree that it should
Speaker:have. And so when people look at how much data they
Speaker:have in an organization and then they think about not only the work it would
Speaker:take to create data governance standards from scratch, but then to
Speaker:retroactively apply those standards to the data they have, it gets
Speaker:very overwhelming very quickly. And so what I would say to someone who is on
Speaker:the fence about implementing data governance is
Speaker:to start small. To start by
Speaker:looking at the key data domains in your organization.
Speaker:So these are the areas like sales Data, marketing data,
Speaker:customer success data, where your organization is
Speaker:producing and or ingesting data about
Speaker:from a high level. I would also tell them to start
Speaker:small by not only defining those key data domains and
Speaker:respective subdomains. For instance, you could have a data domain on
Speaker:sales data and then two subdomains could be inbound and outbound
Speaker:leads and those are two subdomains you can collect data on. But
Speaker:then you also want to apply that data to a particular
Speaker:project that is contained and that has been
Speaker:already greenlit by the sea level leadership
Speaker:as having high value to the organization. I think
Speaker:that does two things. It helps you contain
Speaker:your efforts so that you are not reinventing the wheel
Speaker:across all areas of the organization, and it also
Speaker:ensures that you are working on something that senior
Speaker:leadership really cares about that is also essential. I talk in the
Speaker:book about finding the right sponsor for your data
Speaker:governance efforts, and that really is crucial because like any big
Speaker:transformation, it has to be a top down effort. If you're the Chief
Speaker:Data officer and your C suite, your chief
Speaker:executive officer is not on board with data governance,
Speaker:you can make some progress. Because, again, if you're a senior data leader,
Speaker:your entire job is to strategically manage data as an
Speaker:asset. And so you can make some progress. But without that high
Speaker:level buy in and without connecting your efforts back to the
Speaker:business, you're really going to stall. So I would say start
Speaker:small. Look for a strategic project where data governance
Speaker:can add value, and then do everything you possibly can to
Speaker:connect your governance efforts back to that business goal.
Speaker:So it sounds like someone should write a book about doing
Speaker:data governance from scratch or something like that. That
Speaker:would be a nice idea. It would have helped me on some of my early
Speaker:projects, which is why I wrote the book that's well, I. Was
Speaker:going to lead into that. And you mentioned the book in your answer, and
Speaker:Lauren has written a book for those who are listening, and it's
Speaker:called Designing Data Governance from the Ground
Speaker:Up. And I just picked
Speaker:up the ebook. We were looking at your
Speaker:bio before the show. Frank and I connect about five or six
Speaker:minutes before the show, and I said, that sounds
Speaker:like something I need to dig into. So I picked it up, I'll read
Speaker:it. I've got a little bit of vacation coming up here starting at
Speaker:the end of the month, so maybe I'll get to it then. I'm looking
Speaker:forward. Hopefully you'll read it on the plane there or
Speaker:back. Because I always joke that if someone's reading my book on a beach somewhere,
Speaker:something's gone wrong, because this is not exactly a light hearted beach
Speaker:read. And I always joke with people
Speaker:because when I encounter resistance to the concept of data governance, I
Speaker:joke with them, well, you might not want to read my book, but you're going
Speaker:to have to read the book at some point. So hopefully it will be helpful
Speaker:when you do. I look forward to it. And as we were talking
Speaker:a little in the virtual green room about this,
Speaker:and I said, I'm basically a data
Speaker:engineer. I came into data
Speaker:from software and I made the leap about
Speaker:probably 20 to 25 years ago when
Speaker:a lot of I would call it process
Speaker:control, because before I did software, I was in manufacturing.
Speaker:So it had a lot of the same types of
Speaker:thinking around engineering and process control.
Speaker:And even back then, some of the buzzwords that sound
Speaker:new in software are new ish we were doing in
Speaker:the 90s in manufacturing stuff like Kanban and Six
Speaker:Sigma and those sorts of metrics collection.
Speaker:And I was very fortunate to be trained by
Speaker:someone who was trained by W. Edwards Deming
Speaker:himself on that information. So very
Speaker:fresh, probably some insights that I'll never
Speaker:share, but just interesting to
Speaker:get. Definitely a true believer and someone who came at it with an open
Speaker:mind and really understood it, but
Speaker:these sorts of things that have grown out of that, and I see this as
Speaker:growing out of the data governance is one of the things that grew out of
Speaker:a combination of compliance and quality. Would you agree
Speaker:with that or would you correct me? No, I do agree with
Speaker:that. I think that actually hits the nail on the head. We
Speaker:have let data grow
Speaker:unchecked, broadly speaking, and
Speaker:that is because we just didn't know, as an industry
Speaker:and society how to manage it. You're exactly right that there are people who have
Speaker:been data architects, engineers, scientists for decades, and
Speaker:they've been doing this work for a very long time outside of
Speaker:the public view. But what's different about the work today is
Speaker:the volume of data that is produced by consumer products
Speaker:and the amount of sensitive data that is effectively
Speaker:floating out in the world today through various
Speaker:cloud systems and various products that are used. And
Speaker:to that end, we're now in the earliest stages of
Speaker:figuring out how to manage that from legislative standpoints, both
Speaker:in the US. And abroad. GDPR legislation in
Speaker:Europe comes to mind. That's fairly recent legislation that gives EU
Speaker:citizens a lot more personal rights over their personal
Speaker:data and what organizations can do in terms of profiting from
Speaker:that data. We do not have the equivalent of federal legislation
Speaker:here in the US. But I do see that changing over the next
Speaker:five to ten years. And I think what you also said about
Speaker:quality really rings true. That's a huge issue because
Speaker:we as an industry really lack consistent,
Speaker:clear standards which define what data quality
Speaker:is and how we should be measuring it. And that's a big difference.
Speaker:If you look at fields like medicine law areas
Speaker:that have very high impact on the
Speaker:public, they have pretty clear governing bodies and
Speaker:standards for how doctors and lawyers should do their
Speaker:work. We have things like IEEE, we have
Speaker:the association for Computing Machinery, we certainly have membership
Speaker:organizations where people can get together and discuss these things
Speaker:and debate these issues. But we really lack a
Speaker:clear framework for data quality and
Speaker:compliance, which I think is very long overdue. So
Speaker:I do see that as being the double pronged issue today. And I'm
Speaker:also curious what your take is, as someone who's been doing this work for
Speaker:decades. How have you seen data governance evolve
Speaker:from the 90s through to the present day?
Speaker:Well, it's interesting
Speaker:as I've made the transition from being an employee to
Speaker:being a consultant, which happened around 2005,
Speaker:2006, I definitely saw some difference there.
Speaker:But as an employee at one place, and actually I was a
Speaker:contractor there too, attempt
Speaker:they worked with medical devices. And so there
Speaker:I saw a strict compliance, but it almost fed down
Speaker:from the culture. You mentioned culture earlier as being very important.
Speaker:I totally agree. But it was almost an
Speaker:accidental culture shift that came from the medical
Speaker:device part, the medical part of the medical device field
Speaker:into all aspects of software and
Speaker:data. And it was really interesting to see how
Speaker:that sort of thinking led to
Speaker:almost a practice of data governance. And we weren't even
Speaker:calling it calling it data governance back then, right? We were
Speaker:just considering it software and data. That was
Speaker:all. I fell under that umbrella. And having that experience
Speaker:there was very eye opening and going from there to more of a startup
Speaker:culture, which not picking on startups. There's
Speaker:a priority difference, though, between that and somebody
Speaker:in kind of a more stayed and stable
Speaker:environment. And I'm not picking again, I'm not calling
Speaker:startups unstable. There's a lot of
Speaker:benefits to startups and a lot of
Speaker:innovative cultures, and some of that wasn't
Speaker:present in the more medical device environment. Some of the benefits
Speaker:of that kind of drive and ambition and go, go
Speaker:and get things done. But it's very easy to overlook. And I saw
Speaker:it, I saw important aspects of
Speaker:what we now call data governance and really just good
Speaker:engineering practices. Some of that was overlooked, some of it was
Speaker:deprioritized for what I consider
Speaker:to be mostly legitimate business concerns in a startup
Speaker:world. I would agree with that. I think when you
Speaker:consider startups and the landscape they're in, they
Speaker:have to innovate and be different or else they will not
Speaker:survive in the marketplace. And so their priority really is to
Speaker:move fast and figure it out later. I gave a talk
Speaker:at Data Architecture Online last week and the
Speaker:keynote moderator made a joke about how
Speaker:developers are often like, don't bother me with requirements on
Speaker:coding, meaning they're tinkering and they'll figure it out
Speaker:later. And we've really taken that approach with data
Speaker:and that it's a really tricky balance
Speaker:to balance those standards and the creation of those standards
Speaker:with the need to innovate and stay
Speaker:in business. And that's really what startups are focused
Speaker:on. And then on the flip side, you have these
Speaker:large, highly regulated, highly bureaucratic industries
Speaker:like government, healthcare, medicine,
Speaker:law, which are highly regulated, and they have
Speaker:to exist to be stable and to provide
Speaker:services in a way that their users can rely
Speaker:on. And so innovating, not only is it not the
Speaker:priority in those environments very often, it's also
Speaker:an inherent risk because people in those environments are not
Speaker:really rewarded for doing something in a new way,
Speaker:but they will be very highly penalized if something goes wrong.
Speaker:I think you talked and touched on motivation earlier,
Speaker:and you really have to examine the motivations of whomever
Speaker:you're working with and consider the context. The book that I wrote is
Speaker:a 100 page six step guide to designing your
Speaker:first data governance program from scratch. And it is short
Speaker:enough because there is a lot of nuance when it comes to data governance.
Speaker:When you implement a data governance program for 100,000
Speaker:person multinational firm, that is going to look very different than doing
Speaker:it for a 25 person startup. But the
Speaker:key aspects of governance are the same,
Speaker:I argue, across those nuances. And so that's why the book
Speaker:is short in the first instance, because it's meant to be the first
Speaker:prelude to whatever gets more specific about
Speaker:how to do data governance in your own environment. And that context per
Speaker:environment is really crucial. No, I mean, that's a
Speaker:good point. Data governance, it's come up more and more in my
Speaker:day job as well, because it becomes and it's
Speaker:also interesting. And as the world's imagination is
Speaker:captured by generative AI,
Speaker:I think it's important to realize the generative
Speaker:AI. Well, first off, there's a lot of legal
Speaker:questions that remain unresolved, right? Like, if I tell it
Speaker:to produce a novel in the style of a particular author,
Speaker:andy's laughing because we've been doing some experiments with
Speaker:that. I was muted, but I was laughing. You were
Speaker:laughing. Yeah, more on that later. But no, I mean,
Speaker:what does that mean? If you produce an image in the style of a particular
Speaker:artist, obviously, that is
Speaker:but I think the legislative hammer is coming down on that.
Speaker:And my opinion is it's probably best to start with governance
Speaker:today to save you what a stitch in time will save nine
Speaker:legal bills later. Like something like that.
Speaker:Do you think that generative AI is really going to
Speaker:make the data governance cool, for lack of a better
Speaker:term? That's a really interesting question. I think it is absolutely going to make
Speaker:data governance essential. And I was speaking to somebody on
Speaker:a separate podcast this month about this very issue
Speaker:because you mentioned writing a book in the style of a particular
Speaker:author giving generative AI the prompt
Speaker:to write a novella in the style
Speaker:of cormac McCarthy, for example. In that case, you
Speaker:are maybe not
Speaker:copying or plagiarizing cormac McCarthy's work directly,
Speaker:or maybe you are. It really depends on whether the generative
Speaker:AI can actually understand what you mean, and it can understand
Speaker:cormac McCarthy's style of writing enough to
Speaker:produce a novella in his
Speaker:likeness, if you will. Likeness is a very interesting
Speaker:concept, I think, these days. And you're right, it is incredibly
Speaker:murky from the legal standpoint. And I was speaking on a
Speaker:podcast recently about this in the sense of
Speaker:where when we look at the legal landscape of generative AI, where
Speaker:is there going to be progress? And rather
Speaker:than making progress on the consumer data
Speaker:privacy and consumer rights aspect of the issue,
Speaker:I actually think that we're going to see more progress
Speaker:made and more cases brought to court on the grounds of
Speaker:copyright infringement. If you look at things like
Speaker:using a music in a movie or
Speaker:using images that a corporation owns in a book,
Speaker:I just went through this with my own book. I wanted to use
Speaker:commercial software to make a few diagrams
Speaker:and use templates to do it. And my editor
Speaker:said, are those templates that are pre built into the software? I
Speaker:said, yes. And he said, you either have to get permission
Speaker:legally from their legal department to use those in the book, or you have to
Speaker:create some from scratch and make them yourself. So I chose
Speaker:the latter because it was the path of least resistance. And I think
Speaker:when we consider generative AI and what that means for
Speaker:data, we in the United States are going to see more
Speaker:progress on the grounds of copyright
Speaker:infringement than we are on data privacy and consumer
Speaker:rights in the short term. Now, having said that, I think humans are
Speaker:inherently reactive. And I do foresee
Speaker:in the future, within the next five years, certainly there's going to
Speaker:be a data breach to such a degree
Speaker:that there is going to be enough groundswell for
Speaker:organizations to really get serious about protecting
Speaker:consumer rights and as it pertains to data.
Speaker:The other model you can look at is
Speaker:what's happened in cybersecurity three to five years ago. There were very
Speaker:few conversations happening about being proactive when it comes to
Speaker:cybersecurity. And in recent years, we've seen a
Speaker:large increase in breaches, not just within
Speaker:software companies, not just within organizations, but even
Speaker:breaches of oil and gas pipelines,
Speaker:things like that. And so just like with data governance
Speaker:no longer being a nice to have, it never was to begin with, but now
Speaker:it really is something that you need. Likewise, we're
Speaker:seeing tech teams really prioritize cyber,
Speaker:not just in their pipelines, not just on the technical side, but
Speaker:also creating a more cyber literate workforce. And. I think there's actually
Speaker:a lot that data practitioners can learn from their
Speaker:counterparts in Sizzos to drive the needle on that
Speaker:front. No, that's a good point. I think connecting those dots
Speaker:are important because
Speaker:when the C suite realizes that this isn't a game anymore,
Speaker:when the SCADA drivers got hacked,
Speaker:or when the Colonial pipeline incident happened,
Speaker:I think that realized in obviously a number of ransomware
Speaker:attacks. I think security became very serious, like, oh, wait a
Speaker:minute, this could affect us and it's not
Speaker:optional anymore, or nice to have. Right. And I think data governance
Speaker:is going to follow that same thing. I think
Speaker:that's an interesting take that you have, is that up till now, the only
Speaker:driver in this space has effectively been privacy legislation,
Speaker:right. GDPR probably being the poster child for
Speaker:that. But I can easily see
Speaker:fear of being involved in some massive
Speaker:copyright lawsuit would probably like, I know there's some
Speaker:controversy about how GPT was trained, right? Like he was trained on Twitter
Speaker:data and then Elon Musk said, wait a minute, did you get anyone's approval for
Speaker:that? On that
Speaker:note, I would also encourage people because every now and then I have
Speaker:the strong urge when I am transcribing,
Speaker:for instance, user interviews, to use a tool like chat GPT. It would be
Speaker:incredible if I could feed that video content into
Speaker:a system to spit out an accurate transcript.
Speaker:And that is absolutely not an option for the
Speaker:role that I'm in, for the industry I'm in. I cannot give that proprietary
Speaker:information to anyone outside of my organization. And if
Speaker:I did, the consequences would be things that I don't even
Speaker:really want to think about because I am beholden
Speaker:to keeping that information private. And what
Speaker:that calls to mind is the Samsung incident.
Speaker:Pretty early on in Chat GPT where folks fed
Speaker:proprietary Samsung data to chat GPT.
Speaker:OpenAI owns that now. Again,
Speaker:we as a society, we as an industry don't
Speaker:have the full context or real
Speaker:comprehension of what that actually means, what ownership really means.
Speaker:But on a very practical level, it does mean that highly
Speaker:sensitive commercial data is now with the hands
Speaker:of this very large nonprofit to be used
Speaker:in very different contexts in very different ways.
Speaker:And the consequences of that are really going to be felt
Speaker:and continue to be felt, I think, over the next several years.
Speaker:That's interesting. I was just going to say it's almost
Speaker:like the I'm not sure how accurate
Speaker:it is, but knowing the source I heard it from, it's probably
Speaker:likely that a
Speaker:game manufacturer received
Speaker:proprietary information from a defense contractor
Speaker:in the US. I don't want to get too specific.
Speaker:It sounds like something is hitting the fan and it's not
Speaker:parmesan cheese. Well, it
Speaker:was an argument. The bit that I will share is it was an argument
Speaker:about someone had made a guess about what the
Speaker:interior of some piece of equipment looked like and someone said, no,
Speaker:it looks like this. And they actually
Speaker:supplied documents to prove that. And that wasn't
Speaker:good. Wow. Yeah, that was pretty wild. It was like
Speaker:all on discord server too. Exactly. Which was
Speaker:notoriously secure.
Speaker:So many wrong things about that, yet that happened. It's
Speaker:off the charts. But I mean, it's a good example of good
Speaker:intentions going horribly wrong. And you think that's
Speaker:a thing in data governance as well, like a risk?
Speaker:Absolutely. And when I talk about bias in AI, which is
Speaker:one, I don't believe, again, that data governance is separate
Speaker:from bias mitigation in the training
Speaker:process. I think data governance is a form of
Speaker:risk reduction and bias
Speaker:troubleshooting. And I do think that the
Speaker:overarching issue here is that we
Speaker:really need to think of this as an integrated problem
Speaker:that is one with the business. But I also think
Speaker:that people it's a misnomer to
Speaker:say, of course hackers have nefarious intent in many
Speaker:cases. Of course, there are always going to be people that want to manipulate
Speaker:data, that want to use it to cause harm.
Speaker:There's no doubt about that. But the vast majority of times when we
Speaker:see the biased outputs of algorithms or we
Speaker:see data governance gone wrong, no one was trying to
Speaker:harm someone. There was no negative
Speaker:intent. There are many complicated technical reasons why an
Speaker:algorithm can produce biased outputs towards one user group over
Speaker:another. And this is kind of where when people say, assume positive
Speaker:intent, I think that only goes so far because I
Speaker:don't believe that most developers or data scientists are
Speaker:trying to or executives are trying to harm people by
Speaker:a long shot. They're really doing the best that they can. But if the end
Speaker:result is still that people's
Speaker:rights are being abused, that
Speaker:resumes are getting screened out automatically instead of being
Speaker:given the proper consideration,
Speaker:if those negative results are still occurring, the intent,
Speaker:how much does it matter? But I do think that's an important
Speaker:distinction. Rather than painting the
Speaker:industry overall as a group of
Speaker:bad people with ill intent, I just don't think that's accurate, and I think there's
Speaker:a lot more nuance to it. It's also important, I think, to show
Speaker:that while these challenges are part of the job,
Speaker:they're inherent in the work of doing data today.
Speaker:Whether you're an engineer, a scientist, a governance
Speaker:person, this is part of the job. And so to that
Speaker:degree, it's somewhat inevitable, but it's not
Speaker:unsolvable. There are tactics that you can use to
Speaker:improve your work in this space, and so I don't want it
Speaker:to be a doom and gloom scenario. There are things that we can do
Speaker:as practitioners to avoid a lot of the consequences
Speaker:we're talking about, and there
Speaker:are a lot of blueprints out there for how to do this. Like I mentioned,
Speaker:cybersecurity is doing a lot to
Speaker:educate workforces on how to spot phishing attacks.
Speaker:Things like that if you look at it, governance
Speaker:from a stewardship perspective and a governance council
Speaker:perspective, if you've ever certified on a nonprofit board, nonprofits
Speaker:are actually surprisingly advanced when it comes to
Speaker:things like data governance. When I was writing the book, I found
Speaker:many universities washington University in St. Louis
Speaker:comes to mind that have full websites devoted to their
Speaker:data governance charter, who serves on the governance
Speaker:council, what they manage on it. And I'm sure those
Speaker:people would tell you that their governance council is far from perfect,
Speaker:but they're doing the work, they're holding themselves accountable,
Speaker:and they've set up the structure to succeed. So
Speaker:nonprofits and the cyberspace are both two
Speaker:really strong models to look towards when we're thinking about
Speaker:what the future of data governance looks like.
Speaker:No, that's a good way to look at it. It's an evolving
Speaker:field, and it's
Speaker:interesting how it's finally coming up, and it's becoming more and more
Speaker:prevalent, at least in the conversations I have. And
Speaker:that's encouraging to hear, because like I said, when I was pitching the book and
Speaker:then writing it, I felt confident that this
Speaker:information was necessary, that people in the field
Speaker:could use it. But at the same time, I was seeing
Speaker:relatively little being written about data governance. I was seeing a lot of
Speaker:articles on different things you could do with data from the data
Speaker:science side or engineering side, but I wasn't seeing a lot about
Speaker:governance, and there was that nagging part of me that
Speaker:worried. I feel confident about this book and
Speaker:its subject, and I do worry that it's going to
Speaker:land with a bit of a little thump and
Speaker:then go nowhere. But I've actually really seen the conversation in
Speaker:our industry shift this year. I think it's no accident that that
Speaker:happened when Chat GBT became mainstream, when Generative AI
Speaker:officially became mainstream. And that really was
Speaker:my thought all along, was that we were going to reach a
Speaker:tipping point where data governance was necessary. And so I would even
Speaker:go so far as to say when the book was in beta last fall, I
Speaker:still had some of those concerns about whether it was going to be
Speaker:relevant enough or perceived to be relevant enough, and
Speaker:I don't have that doubt anymore.
Speaker:So it's interesting. I see that there's an Audible version too. That's
Speaker:awesome. There is. And so they did turn it into an audiobook. So if
Speaker:people want to read it, they can either pick up an e
Speaker:copy, which is available on any ereader, they can also
Speaker:order a print copy, but it is also available on
Speaker:audiobooks. So if people want to utilize that I know
Speaker:that audiobooks are preferred for people on the go. I listen to
Speaker:them at the gym or on planes, and so I
Speaker:find that audiobooks can be a great
Speaker:alternative. If you don't have that time to sit and read every
Speaker:day, you probably at least are sitting down at some point during the
Speaker:day, whether on a commute, whether on a plane. And so hopefully the audiobook
Speaker:can help. No, absolutely. Because
Speaker:of circumstances related to what I mentioned early
Speaker:in the show about the good news, I was just spending a lot of time
Speaker:in the car between here and Pittsburgh. So I've gotten a lot of audio
Speaker:books done in there and I think this is an
Speaker:awesome conversation. This could probably go on for the 2 hours, but I want
Speaker:to switch to the pre canned questions. But while
Speaker:hopefully Lauren, you've had a chance to review those before. Oh, Andy
Speaker:just posted them, it looks like. Well, let me post them over
Speaker:here in our team's chat. Oh, I just did
Speaker:it. They're not brain teasers,
Speaker:but they're just fun little questions that we have, we ask of every guest.
Speaker:But I will point out that Audible is a sponsor
Speaker:of Data Driven, and if you go to
Speaker:thedatadedrivenbook.com, you could pick up a free book.
Speaker:And I'm looking forward to listening to your book. Lauren.
Speaker:Awesome. Thank you so much. That really means
Speaker:excellent. Yes. And if listeners want to
Speaker:buy the book, you can go to Pragueprog.com. That's
Speaker:Pragprog.com. The book is
Speaker:called Designing Data Governance from the Ground Up, and your listeners can
Speaker:use the code Datagov 23 all
Speaker:Caps to get 35% off the e copy.
Speaker:So if folks are interested and they need a little bit of a
Speaker:boost, that code should be good, and I
Speaker:would love to know what folks think. So I'm happy to be connected with on
Speaker:LinkedIn and if folks want to leave reviews of the book on sites
Speaker:like Amazon and Goodreads, that is also hugely helpful.
Speaker:Those reviews really do make a difference in books getting found and
Speaker:discovered on those platforms, so every review helps.
Speaker:Awesome. All right, our first question. How did you find
Speaker:your way into Data? Did you find Data or did Data find you?
Speaker:Data did find me. I'm a writer at heart,
Speaker:and I have a background in mixed methods
Speaker:research, journalism, and digital media and
Speaker:content management. I started using open source CMS
Speaker:systems to manage that content. So that's my
Speaker:first foray into open source tech and communities. But I
Speaker:didn't really get interested in Data until I was a research analyst at
Speaker:Gartner and I started learning about AI
Speaker:that way. That's where I started hearing about different types of AI,
Speaker:things like natural language processing versus robotic process
Speaker:automation and how you could use these different types of tech to
Speaker:solve very specific business problems. And I was
Speaker:surprised by how interesting I found
Speaker:that whole aspect of it and how interesting I found the fact that at
Speaker:the end of the day, AI is data, and the more
Speaker:you learn about data and the more you know about it, the more you can
Speaker:use those technologies effectively.
Speaker:Awesome. You want to take the next question, Andy?
Speaker:Yes, sure. Sorry.
Speaker:I was thinking of how that parallels Frank's story a little bit.
Speaker:I beat Frank up about this every chance I get because I
Speaker:begged him for, like, ten years to come over to
Speaker:data and specifically analytics and business
Speaker:intelligence because Frank is a gifted natural
Speaker:artist. He's one of those people that can draw.
Speaker:And I'm almost 60 years old. I still can't
Speaker:color in the lines. So I had to do something like data engineering
Speaker:that didn't require that artistic bend.
Speaker:But I was thinking of that, as you mentioned, that could I use this
Speaker:to beat Frank up and see, I did
Speaker:it's in love. Frank, you know that. Oh, I totally know. I totally know.
Speaker:Yeah. It only took the collapse of Silverlight
Speaker:and Windows Phone for me to see the light. I'm so sorry that
Speaker:happened. That's okay. Our second question.
Speaker:Lauren, what's your favorite part of your current gig?
Speaker:My favorite part of my current gig is talking
Speaker:to users of a particular product. And
Speaker:when the light bulb goes off between what they're saying
Speaker:is a pain point and a possible solution that we can build or
Speaker:design, that gets really exciting to me. And
Speaker:so you can get a little overwhelmed by all of the user interviews
Speaker:that you do, especially in the beginning when you're taking in a lot of information.
Speaker:But then as you zoom back and then start looking at the big
Speaker:picture to see how you might solve some of those
Speaker:challenges with technology, that's where I see the
Speaker:real clear overlap between those user interviews and
Speaker:what is designed and put out into the world through tech. And
Speaker:that's really exciting to me. Got you.
Speaker:Our next complete the sentences when I'm not working. Well, we have
Speaker:three questions sorry, too much coffee. We
Speaker:have three questions that are complete the sentence. Right. So the first one is, when
Speaker:I'm not working, I enjoy blank. I enjoy
Speaker:traveling. I love to travel as much as my time
Speaker:and money allow. And one of the cool things about working in Tech is that
Speaker:you get to attend a lot of conferences that are in really cool places. So
Speaker:by virtue of being in Tech, I've gotten to see a lot of
Speaker:new cities and even some countries in places.
Speaker:For instance, I'm scheduled to go to North
Speaker:Macedonia next month to help teach at a tech
Speaker:camp in Orid, North Macedonia. And I would not
Speaker:be going if not for my career in Tech. But I love
Speaker:to explore new places, and doing that is one of the few things that actually
Speaker:gets me to turn my brain off, and that's one of the things that I
Speaker:value about it. So I do that as much as time and money
Speaker:allow. I am with you. Yes. I like to not
Speaker:look at a calendar. That's kind of my thing. Yeah.
Speaker:And it's a luxury in this day and age, and when I get
Speaker:to do it, that's really special Macedonia.
Speaker:I've never been into that part of the world and I am jealous.
Speaker:Yes, I'm looking forward to it. Other than Croatia,
Speaker:I haven't been to the Balkans. I've seen very little of Central and
Speaker:Eastern Europe as a region. And that's the thing about travel. As much
Speaker:as you've seen, there's always more to see and you know that
Speaker:you can't possibly scratch the surface of all of it. So I really
Speaker:value every opportunity that I get to see something new.
Speaker:Excellent. So our second complete the sentence is I think the
Speaker:coolest thing in technology today is blank.
Speaker:I think the coolest thing in technology today
Speaker:is the opportunity to
Speaker:get time back to plan more
Speaker:effectively. And so that might sound like a catch
Speaker:22, but I think when we look for opportunities to
Speaker:automate really repetitive tasks that take people hours,
Speaker:if not days to complete, it does give you a lot of
Speaker:time back to be more strategic about how you complete
Speaker:the essence of your work. And so one example of that is I teach a
Speaker:course on interaction design at George Washington University and I had a student this past
Speaker:semester ask me about the
Speaker:impact that I think AI will have on the design profession. And I said,
Speaker:well, you're already using AI and design today because it's embedded
Speaker:into Canva and mural and all of the
Speaker:software that you use to make these designs. And you're
Speaker:already pretty adept at using AI, but what it can't do
Speaker:is teach you to get really granular about the best
Speaker:way to design that technology to
Speaker:do a particular task that can solve a user need. And
Speaker:so I think that that is what's really cool. I think
Speaker:that is what is not easy to be easily automated.
Speaker:And I think that if we can use technology to do
Speaker:the dull stuff, for instance, using natural language processing to comb
Speaker:through hundreds of documents and get you the information you need within
Speaker:minutes, that is on the surface kind of boring,
Speaker:but it's also hugely valuable. It's better in many cases than
Speaker:what humans can do and it gives you more time back.
Speaker:Good answer.
Speaker:Oh, you're on mute, Frank. Frank, I'm on mute. Sorry,
Speaker:but I was coughing. The third and final complete the sentence is I look
Speaker:forward to the day when I can use technology to blank
Speaker:to drive. I would really love. I
Speaker:grew up learning to drive in the suburbs of Boston and then I moved to
Speaker:Washington DC. Which means that driving is not a fun
Speaker:experience for me. And I do look forward to the
Speaker:day when the technology for self driving cars is advanced
Speaker:enough that I can use it to just get in the
Speaker:car, have it drive for me. I
Speaker:do not know what exactly that looks like beyond this idea that I just
Speaker:shared because obviously self Driving Cars and Regulation
Speaker:is a whole other podcast. But I do look forward to the day
Speaker:when, like, planes being effectively flown on
Speaker:autopilot today. I do look forward to the day when we can actually do that
Speaker:with cars. I wholeheartedly agree on
Speaker:that one. Driving in there's something about driving in and around
Speaker:DC that is just an unpleasant experience. It is. And it's gotten
Speaker:worse over the pandemic, for sure. I notice a lot more speeding,
Speaker:a lot more people running red lights, a lot more people going through intersections.
Speaker:And as someone who straddled the border of DC and Maryland
Speaker:for seven years, maryland drivers are truly terrifying.
Speaker:And so I hope that self driving
Speaker:cars can alleviate a lot of that. As a Maryland resident,
Speaker:I do not disagree. I was
Speaker:just going to interject that here in Farmville, Virginia. It's tough, too. I
Speaker:mean, just the other day there were like five cars at the light.
Speaker:It's a rough one. The struggle is real,
Speaker:by the way. I agree with self driving, even though it's all
Speaker:rural around me. Share something different about
Speaker:yourself, Lauren. But we remind all of our guests
Speaker:that we want to keep our clean rate. Yes. So
Speaker:something different about me is that I foster
Speaker:dogs. So I have a dog myself. I have a
Speaker:rescue dog who is my little work from home buddy.
Speaker:But I also foster dogs every now and then. And so I fostered
Speaker:a total I did the math recently. I've fostered a total of
Speaker:ten within the past two years. And so every now and then
Speaker:I have two pups at home, and I always encourage people to
Speaker:foster whenever I can. We're in the summer right now.
Speaker:Summer is a notoriously busy season at Shelter. So
Speaker:if you have ever considered fostering a
Speaker:dog, a cat, any other animal that just needs a home to
Speaker:decompress in before they get adopted, I highly recommend that people
Speaker:consider it. That's cool. My wife and I have done the
Speaker:same, and we've only managed to keep two.
Speaker:Yeah, well, so one of them I did end up adopting. I did
Speaker:adopt one foster, but the others and
Speaker:people say they're like, well, is it hard to give them up?
Speaker:And it is to some extent, but I also think,
Speaker:you know, when you're a stop on their journey versus
Speaker:their final destination and it's hard to
Speaker:explain it more than that, but it is a gut feeling. And
Speaker:so I think you actually know, like I said,
Speaker:I highly encourage people to do it. The way I also sell it to people
Speaker:is you get all the fun of having a pet around without
Speaker:the bills and long term responsibility. So
Speaker:that's also good if you just want a little buddy for a while
Speaker:but don't want a pet long term, that works out, too. It is a bit
Speaker:like Uber for dogs in that sense, or whatever animal.
Speaker:Yeah, no,
Speaker:we had a whole litter of puppies once that were fostered with us, and it
Speaker:was really cool to have that little baby puppy experience,
Speaker:but. Yeah, it sounds like a lot of work, though.
Speaker:It was. And then as they got adopted, I was like, okay,
Speaker:yeah. I'm happy to see them go to their new homes where they're the center
Speaker:of attention.
Speaker:That's part of the justification for moving where we did now, where we have like,
Speaker:four acres, was for the dogs, basically. I work hard
Speaker:so my dog has a better life. Oh, totally.
Speaker:I work to support my dog. At the end of the day,
Speaker:we have a dog, but we're owned by five cats. Share it
Speaker:on. That's also a good way to put it. Yeah. You're including
Speaker:the dog. The dog is also owned by the cats, I'm guessing.
Speaker:And our final question, where can people find more about you and what you're
Speaker:up to? Yes. So I am active on LinkedIn, so
Speaker:if people want to connect to me, I would welcome that. I'm on there under
Speaker:my full name, and then they can also, like I
Speaker:mentioned, go to Pragprov.com to find the book.
Speaker:So that would be fantastic if your listeners want to find it and
Speaker:download it and then let me know what they think. So those are the main
Speaker:avenues. I am on Twitter as well, although less so
Speaker:these days. And I am trying out new
Speaker:platforms like Threads. I'm active on
Speaker:Instagram already, and so I did decide to
Speaker:try out Threads as well. That is TBD, but that's used
Speaker:in more of a personal context. I don't talk to my friends
Speaker:about data governance in my everyday life, but that's also partially why I like
Speaker:talking to people like you about it. Cool. Well, thank you. And
Speaker:with that, we'll Let Bailey, our AI
Speaker:assistant, and the show. Thanks for joining us.
Speaker:Thank you, guys. Thanks for listening to data driven
Speaker:have you checked out Data Driven magazine yet? We are looking for
Speaker:writers for the Autumn 2023 issue. Please check
Speaker:out Data Driven magazine.com for more information. Thanks
Speaker:for listening, and be sure to rate and review us on whatever podcasting app