Inna Tokarev Sela on Approaching Data Challenges with Generative AI
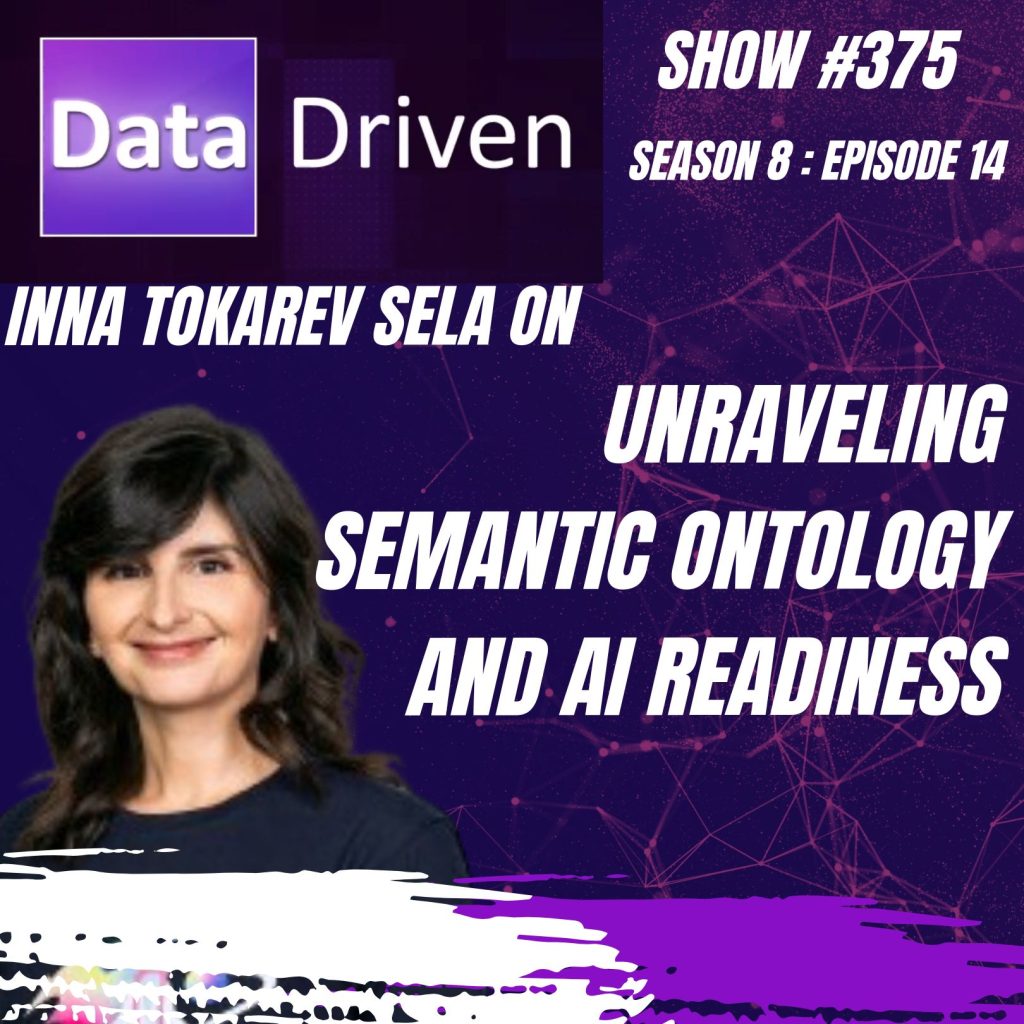
Welcome to another episode of “Data Driven,” where we dive into the ever-evolving world of data science, AI, and data engineering. Today’s special guest is Inna Tokarev Sela, CEO and founder of Illumix. Join hosts Frank La Vigne, BAILeY, and Andy Leonard as they unpack Inna’s groundbreaking insights into generative AI, the future of data management, and the intricacies of AI cost effectiveness.
Inna reveals the origin of her company’s name, “Illumix,” and discusses the pressing risks of 2025, particularly the total cost of ownership for managing generative AI. She highlights the inefficiencies of data customization and proposes a shift towards moving AI closer to the data to reduce costs. Through the unique lens of Illumix’s approach, Inna explains how they aim to illuminate organizational data by using a virtual semantic knowledge graph based on industry ontologies and business logic.
Timestamps
00:00 Ina Tokarav Sala: CEO of Illumix, AI readiness pioneer.
05:57 ROI and data are crucial for decisions.
08:56 Intermediate stage: copilots, insights, static dashboards persist.
11:12 Illumax targets structured data market, unlike others.
14:29 Bad data skews predictive analytics, causing errors.
19:48 Data modeling efficiency increases with virtual assistants.
22:33 E-commerce evolution: convenient online shopping preferred.
25:27 2025’s biggest risk: High generative AI costs.
27:07 Focus on domain knowledge and metadata utilization.
31:44 Predicting patterns is profound, not crazy.
36:09 Industry trends are cyclical, like fashion trends.
37:49 Repatriating data due to AI cost efficiency.
40:47 Data processing everywhere raises security concerns.
45:00 Founder freedom: Experimentation unlike SAP’s structure.
49:11 I’m considered controversial for being very visionary.
52:29 Truth’s evolution parallels past technological shifts.
54:39 Frank’s World: Kids show on recycling, BBC.
57:09 Thank you, Ina Tokarev Saleh, for insights.
Transcript
Welcome back to Data Driven, the podcast that peeks into the
Speaker:rapidly evolving worlds of data science, artificial intelligence,
Speaker:and the underlying magic of data engineering. Today's guest
Speaker:is someone who's redefining the rules of the game in AI and data,
Speaker:Ina Tokarev Saale. She's the CEO and founder of
Speaker:Illumix, a company pioneering the use of generative semantic
Speaker:fabric to make organizations AI ready. We'll dig into how
Speaker:Ina's background as a frustrated data user sparked her innovative
Speaker:journey, why 80% of enterprise decisions still aren't data
Speaker:driven, and her bold vision for a future with app free workspaces
Speaker:where AI copilots handle the heavy lifting. Oh, and we're
Speaker:tackling the ultimate question. If the future is already here,
Speaker:why does it still feel so delightfully chaotic? Sit
Speaker:back, grab your favorite coffee mug, or a Maryland state flag
Speaker:one if you're feeling fancy, and let's dive in.
Speaker:Alright. Hello, and welcome back to Data Driven, the podcast where we explore the emergent
Speaker:fields of data science, artificial intelligence, and, of course, it's all made
Speaker:possible by data engineering. And with me today is my most favoritest
Speaker:data engineer in the world, Andy Leonard. How's it going, Andy? It's going well,
Speaker:Frank. It always warms my heart when you introduce me like that. Well, you are
Speaker:my most favorite data engineer. Well, that's cool. You're well, you're my
Speaker:most favoritest. I like, there's so many things. Right? Data
Speaker:scientist, developer, evangelist.
Speaker:I mean, there's all sorts of cool things that you do. Super,
Speaker:certified person. What are you up to in certifies in certification?
Speaker:12. Wow. Yeah. I'm in I'm in the
Speaker:New York City area code now. So that's good. Next
Speaker:up, the Bronx area code 718. So Wow. That's a
Speaker:big jump. Yeah. Yeah. We're we're working on we're working on it, and I'm at
Speaker:760 some odd consecutive days. I'm at the point now
Speaker:where when I post anything on about Pluralsight
Speaker:or, my number, the search or the number of
Speaker:days, Pluralsight always sends me a congratulations, Frank. Keep
Speaker:going. So, like, I'm on their radar now. So which is really
Speaker:nice. I don't know. It's super cool. Yeah. It is super cool, which reminds me
Speaker:I still have to do 2 days. But in the
Speaker:virtual green room, we were talking about coffee mugs. We
Speaker:were. And, we're we're I don't have a coffee mug with
Speaker:me today, but, there's an
Speaker:interesting anecdote from a previous show, which I think the show is live now, about
Speaker:the Maryland state flag coffee mug, which is, pretty funny.
Speaker:So today we have with us a very special guest,
Speaker:Ina Tokarav Sala. She's the CEO and founder
Speaker:of Illumix, and a pioneer
Speaker:of generative semantic fabric, which I wanna know more about that, but it
Speaker:empowers organizations with AI readiness throughout her career
Speaker:leading data products, monetization, and as a data
Speaker:stakeholder. Ina recognized the oxymoron of our
Speaker:domain. Despite huge investments in data and analytics,
Speaker:most business decisions are still not based on these data or
Speaker:insights. And when I read that, I felt that one.
Speaker:So she, she works she founded this company,
Speaker:Lumix, which is, the the byline says, get your organization
Speaker:data generative AI ready. So So welcome to the show, Ina.
Speaker:And, tell us about this. Like, because I think this is a big problem
Speaker:with generative AI. Well, first off, let's tackle the big
Speaker:one, which is the idea that despite all this money that's been
Speaker:thrown at data and analytics for at least 2 decades, probably
Speaker:longer, a lot of decisions are not data driven.
Speaker:Yeah. Fine. Can you hear me? Because
Speaker:I see a little bit Yeah. We can hear you. Okay.
Speaker:So yeah. Thank you. You're totally right. The the benchmark says
Speaker:only 20% of decision making in enterprise is based on data.
Speaker:And to me, I I have been around for a
Speaker:while. So 25 years in data analytics, and it was
Speaker:always about cloud, big data. But
Speaker:what it actually boils down to? Are you able to
Speaker:pull out whatever analysis of data you need when you have, like, question on
Speaker:hand? Not really. And this is a situation in majority
Speaker:of enterprises, right? Even if those huge data
Speaker:teams and huge investments in infrastructure and all of that.
Speaker:And to me, the biggest promise
Speaker:of of LLMs in enterprise setting is to
Speaker:to bring the contextual and relevant data
Speaker:to the stakeholders in need.
Speaker:Right? In this experience which is impromptu which
Speaker:means it's improvised, it's governed and hallucination free, it's
Speaker:transparent. So I I would totally love have to
Speaker:have this experience where I'm in my Slack or Teams, right, and
Speaker:I've been able to to chat with my data copilot
Speaker:and ask a question and get the answer I can base decision happen.
Speaker:Right? Not just an answer. I should be reverse engineering
Speaker:with, you know, bunch of people.
Speaker:Interesting. Interesting. But I don't think that I think that
Speaker:the companies, they
Speaker:they they they throw a lot of data. They store a lot of data. They
Speaker:analyze a lot of data. But a lot of at the end of the day,
Speaker:not all decisions, but a lot of decisions are not based on just the direct
Speaker:decision of the data. They're based on quite frankly a lot
Speaker:of it's particularly the higher the, higher the
Speaker:level. Sometimes it's based on what's good for the person, not
Speaker:necessarily the organization or the business, let alone the customer.
Speaker:Do you think what are your thoughts on that? I'm familiar with the saying,
Speaker:if you touch your data long enough, it will confess. That's
Speaker:right. It goes exactly to the domain.
Speaker:So I guess you can you can massage the results
Speaker:right? But, secondhandly, when an
Speaker:employee comes to me with suggestion with a business plan with,
Speaker:you know some project I always ask like what's the ROI like what's
Speaker:it going to be to spend and what's the impact on on you know
Speaker:other activities and and what it's going to be on expense of
Speaker:so having numbers having data to you
Speaker:know to the basic decision or to bring to your boss is
Speaker:always has been a struggle and it's still struggle today so I
Speaker:think it overweights maybe some you know,
Speaker:reluctance to have open data for all just for the
Speaker:sake of of being able to to have specific context on it.
Speaker:Interesting. That that is very interesting. And, you know, that I
Speaker:think that's been the the purpose of a lot of
Speaker:data driven activities in in corporations globally
Speaker:is, you know, and for a very long time is how do you convert
Speaker:data in its raw natural form into
Speaker:information? Mhmm. And, you know, and and
Speaker:defining information as, something I
Speaker:can glance at and know, you know,
Speaker:almost instantly how my enterprise is performing.
Speaker:And that was kind of my opening line 20 years ago when I
Speaker:started in data warehousing is to go talk
Speaker:to a decision maker, CIO, CEO,
Speaker:and, you know, try and do a very small, project,
Speaker:a phase 0. And just ask them that, how do you know?
Speaker:And the surprising answer, yeah, even then it was surprising,
Speaker:was, you know, something along the lines of, well,
Speaker:people email, information to
Speaker:a lady out front or a secretary assistant guy out front,
Speaker:and he or she compiles it and puts it into this summary,
Speaker:and then they tell me. And so, you know, 1 PM
Speaker:every day or, you know, Monday on 1 PM. I know how we
Speaker:did last week. Something like that. It's very
Speaker:manual processes. So does
Speaker:does Illumix, address that? The
Speaker:manual part? Yeah. Yeah. Totally. So
Speaker:I don't think reports will go anywhere, but I think we'll
Speaker:have, you know, at least 3 types of
Speaker:experience with data. So I do I do believe in
Speaker:application free future where you have a
Speaker:question or a task and then you have a launcher and you
Speaker:just, you know, articulate whatever request you have.
Speaker:And in the background whatever applications, workloads, and data have
Speaker:been engaged with each other to to basically come up with the
Speaker:results. Right? So I do believe in this future. Right? So this is
Speaker:the ultimate. Right? But I think we will have this intermediate
Speaker:stage where we'll have a lot of copilots or
Speaker:assisted insights in, in the context of
Speaker:applications you're already using. So using your CRM systems, you will have
Speaker:all kind of insights, suggestions, you know, data driven,
Speaker:actions which which might come up with the system in your
Speaker:workflow inside your context. Right? And you might have to have
Speaker:this pure experience when you do go to analytic systems like BI
Speaker:or something else where you do have your static dashboards,
Speaker:day after day, same way that I go to, you know, to to my
Speaker:CRM dashboards and see how pipeline is going and all of that. So I do
Speaker:not them need to them to change. Right? I don't want to go to some
Speaker:chatbot and and ask again and again the same question, like, what's the pipeline
Speaker:conversion today? Right? I do want to have those static dashboards where I just,
Speaker:you know, sneak peek and see if everything in line and
Speaker:we we in the benchmark. So those three types of experiences, I
Speaker:do not think they're going to to evaporate in
Speaker:the future. Right now, we are mostly bound to the last type of
Speaker:experience of being in the closed garden of our BI tools,
Speaker:like this 3 modeled analytic experience and then we'll have this
Speaker:phase where we do have embedded experience. Majority of the companies are
Speaker:already suggesting some kind of improvements in the
Speaker:space, some better, some halfway, let's
Speaker:say. And and the ultimate goal is to to have this
Speaker:launcher when for for majority of ad hoc
Speaker:task of questions, you will have this improvised experience.
Speaker:So a follow-up on that. You mentioned Copilot, and,
Speaker:Microsoft has been the company that I've heard using that term most
Speaker:often for some sort of digital assistance. It
Speaker:to me, outsider looking in, although I I use the
Speaker:tools, it it seems to have been a quantum leap,
Speaker:this year in that technology. It just seems like last year, they were
Speaker:talking about things that it might help with, and I've seen
Speaker:all sorts of examples of this. But have you seen that? Has that been
Speaker:your experience that in the last 12 months, these type of
Speaker:assistants have just, you know, taken a giant step forward?
Speaker:Mhmm. I will address this question together with the previous one, like, how
Speaker:Illumax is is positioned in in this context. So I
Speaker:do see many projects in the companies
Speaker:which, and mainly, they're providing
Speaker:copilots, for call centers or support centers
Speaker:and mainly based on document summarization.
Speaker:Right? So document summary is more,
Speaker:lightweight and and risk averse use
Speaker:of LLM technology where I can actually go and check the document
Speaker:itself based on the resource. Right? So it's kind of and documents
Speaker:are already articulated with lots of context in
Speaker:business language. So it's kind of low hanging fruit and majority
Speaker:of the companies go to the direction including, Microsoft.
Speaker:Where Elamax goes Elamax actually,
Speaker:tackles the market which is less,
Speaker:less digested, the market of structured data. So you mentioned you
Speaker:started your career in warehouse and, so warehouses,
Speaker:databases, data lakes, business applications such as supply
Speaker:chain, ARP, CRM, and all of that. All of that
Speaker:con defined as structured data space. And despite the
Speaker:name, it couldn't be less structured than it is at the
Speaker:moment. Right? So you have If it is structured, it's not structured
Speaker:the way you need it. Yeah. Exactly. So the nay namings are not meaningful, like
Speaker:abbreviations, frank table, or for like abbreviations,
Speaker:the, frank table or and this
Speaker:transformation or alias. Right? So all those weird names especially under
Speaker:SAP systems. I love that and and no
Speaker:single source of truth. Right? In documents, you might have versions, but you do
Speaker:still have some alignment to single source of truth. In data, you
Speaker:can have many definitions even in the same
Speaker:data source. And the thing is, if you put semantic
Speaker:models like semantic search on top of them and it works by proximity,
Speaker:you might have hallucinations and random answers every time you engage
Speaker:with the tool. So this this is where we chose with
Speaker:Illumix to to tackle the problem as,
Speaker:basically, defining as a 3 step approach.
Speaker:Right? The first step is getting data AI
Speaker:ready. So there is no yeah. There is
Speaker:no way of using generative I or AI analytics in general
Speaker:if you do not have other data. But for analytics, which is
Speaker:served to you as BI dashboard, it's actually feasible to do
Speaker:manual data massaging. Right? Well, fun. Yeah.
Speaker:Yeah. That's fun. That's near and dear to my heart as a as a data
Speaker:engineer, data quality. Because
Speaker:you can have the, you know, the fastest, best presentation, the
Speaker:slickest graphics, and it could be totally lying to
Speaker:you. And back, you know, even from the days of of
Speaker:data warehousing all the way through today's semantic models and
Speaker:dashboards, it's a the the quality
Speaker:of the data store you're reporting against,
Speaker:That that data quality, if you were to measure it, you know, there's a number
Speaker:of ways to do it. But it's well north of
Speaker:99% of that. And people see that, and they go, wow.
Speaker:That that's super good. And it's like, no. No. It didn't. You can't do
Speaker:predictive analytics off of something that's 99%
Speaker:because that that 1% of bad data or
Speaker:incorrect data or duplicate data will skew your results.
Speaker:And what often, you know, the the layperson doesn't understand
Speaker:is that if it lies to you and tells you you're gonna make a $1,000,000,000,
Speaker:that's just as bad as it telling you you're only gonna make a
Speaker:$1,000,000 if the if the truth is you're gonna you're at about 25,000,000.
Speaker:That's your real projection if you were to follow that line out and do the
Speaker:extrapolation, you know, properly. And you can make
Speaker:bad decisions with an overestimation just as easily,
Speaker:maybe more so than if it's an underestimation. Yeah.
Speaker:Exactly. So this goes to to, to the ground truth of
Speaker:your results as good as your data is. And you cannot
Speaker:trust, simple semantic search
Speaker:to solve all these problems for you. And
Speaker:so for us, the baseline, the first use
Speaker:case is to get data AI ready or generative AI ready And we
Speaker:do use generative AI for that from day 1. We actually generated company
Speaker:from:Speaker:to explain to our investors back then what it actually means.
Speaker:Yeah. You know, I I get it. I mean, if you build on a crooked
Speaker:foundation, you you can't get anything straight, you know,
Speaker:out of that. So that makes perfect sense to me. And it and,
Speaker:please correct me if I'm mischaracterizing, the work that Illumix
Speaker:does. But is it automated,
Speaker:AI automated, data quality? Is that really what you're
Speaker:after? So, basically, we automated full
Speaker:stack of LLM deployment for structured data, and it takes the
Speaker:AI readiness part. AI readiness, which means we have automated
Speaker:reconciliation, labeling, sensitivity tagging Okay.
Speaker:Like lots of lots of data preparation which is automated.
Speaker:Gartner actually named us as a call vendor for that lately. We have
Speaker:this layer of a context automation. Right? So so any
Speaker:LLM, any semantic model needs context and this context and reasoning
Speaker:usually rebuild by data scientists. To me, it's controversial
Speaker:because, you know we had data modelers which didn't
Speaker:understand business logic and now we have data scientists who do not necessarily
Speaker:fully understand business logic and the model into black
Speaker:box experience of context. Right? So ElamX
Speaker:reverses process. We actually automate context and we wrap it
Speaker:up in augmented governance workflow so business people or
Speaker:governance folks can actually certify it. So it's auto generated
Speaker:context for LLMs but certifiable by humans. We do
Speaker:believe that we need to bring human to the loop, right, to to certify
Speaker:it. Yeah. And the last I love I'm sorry. I have
Speaker:interrupted you, like, 3 times now, and I apologize. I haven't met 2. I
Speaker:thought you paused. So finish please finish your thought.
Speaker:No. No. I'm saying, like, 3 parts. So you already did data governance and the
Speaker:actual alarm deployment because you need to interact with the whole thing, and the interaction
Speaker:to have to has to be explainable and transparent. You need to understand
Speaker:how, especially on structured data, you need to understand how
Speaker:the question was calculated based, sorry, how answer was
Speaker:calculated based on questions and how, data was
Speaker:actually sourced, what's the lineage, what is the governance and access
Speaker:control through search your clients. So all of that should be on the interaction layer.
Speaker:So AI readiness, governance, and the interaction layer explainability to
Speaker:the end user. Absolutely. Okay.
Speaker:Thanks. And I do apologize again for the
Speaker:interruption. So my my characterization of it as something that's just
Speaker:data quality is is way low. There's a little bit of overlap between
Speaker:data quality and what you're describing. You're talking about taking this into
Speaker:that next level that is specific to, generative
Speaker:AI and perhaps other, you know, AI related,
Speaker:AI adjacent technologies, machine learning leaps to mind and stuff like
Speaker:that. But your the tagging, the categorizing,
Speaker:and all of the things you're describing there, that is next level.
Speaker:And it's very interesting to me that you're
Speaker:using AI to get data ready for AI.
Speaker:That's an interesting combination. Mhmm. It makes sense, though. Right?
Speaker:You can kinda scale out human capability with AI. I
Speaker:think that's you you kind of alluded that with Newman in the loop. Right? Like,
Speaker:I think I think where you were kinda going with that, again, don't wanna speak
Speaker:for you, but it's like the idea that AI isn't gonna replace
Speaker:humans. It's just gonna make humans more productive. Yeah.
Speaker:For sure. Augment us because frankly speaking, no one
Speaker:wants to to model data, you know, as their
Speaker:career. We want to solve problems. Right? And to solve
Speaker:problems, we we have to to understand what the problems are
Speaker:And letting AI to surface the problems as alerts and for us
Speaker:to to resolve them as conflicts takes, you
Speaker:know, 1% to 10% of the time that it should take,
Speaker:where we are busy, you know, wrangling data still. And, you know,
Speaker:it's sad to some extent because data is growing and we cannot keep up.
Speaker:No. That's a good point. Even if even if there are people out there and
Speaker:some of our listeners may really do like modeling data. Right? But, you
Speaker:know, Dow, they can model about 10 times the amount of data or maybe
Speaker:a 100 times more. Right? And then ultimately, the expectation of
Speaker:what a, you know, what a person
Speaker:can do in a set period of time is gonna go up just
Speaker:because I I I think I think you're on to something there. Plus,
Speaker:I also I would also, like, double click on the idea that you said earlier,
Speaker:which I think was very intriguing, was this notion of
Speaker:a lot of the apps that you use would kind of fade away. You just
Speaker:have this virtual assistant. You know, I I think back to
Speaker:there's a number of scenes in, you know, Star Trek The Next Generation where they
Speaker:have a conversation with the computer. Right? Mhmm. You know, you they
Speaker:don't they use the computer. They get stuff done. There's no
Speaker:Microsoft Word. There's no PowerPoint. Right? Like, there's no, like, it's
Speaker:just the the there is no application. The application is kind of invisible. It
Speaker:becomes the computer. And I think that's a very
Speaker:intriguing kind of way. And if you had told me that a year ago, I
Speaker:would have been very skeptical. Now I look at it, I'm like, I
Speaker:mean, it's it's it's almost inevitable.
Speaker:Yeah. Yeah. I agree with you. Futures here,
Speaker:it's not evenly distributed as people say. So I
Speaker:guess, you know, when you're attending conferences in Bay Area,
Speaker:it's already it's already here. It happens. Right
Speaker:and when you go to let's say Europe we
Speaker:even just say you know just say a EU act in
Speaker:Europe is is ramping up so it's all about
Speaker:controls and and this is great So I do not think that regulation and
Speaker:innovation, actually, jeopardize each other. I think
Speaker:they should go hand by hand and, that's where I see
Speaker:industry is going. So so East Coast approach, majority of our customers
Speaker:are coming from East Coast US, Pharma,
Speaker:financial services, insurance, highly regulated data
Speaker:intensive companies. They have now,
Speaker:sometimes even inventing standards for generative AI
Speaker:implementations because everything is so new but companies
Speaker:want to go fast. Right? So no one wants
Speaker:to to downplay risks on one hand. On the other
Speaker:hand, everyone want to, you know, to implement generative AI
Speaker:and see the productivity cuts. It's, you know, it's evident productivity
Speaker:cuts are already here with all those co pilots summarization,
Speaker:what have you and this is where we are today. So I
Speaker:think like again Bay Area running fast
Speaker:and east is coming up with regulation. We will meet somewhere
Speaker:in between. I believe in both. Well, if you kind of,
Speaker:like, look at, like, historically, you know, when .coms first
Speaker:started, right, there were a number of, hey. Look. You know, we're gonna sell pet
Speaker:food online. Right? Like, and then it was
Speaker:like, back in the dial up days, it didn't really make a lot of
Speaker:sense. So it would just be easier for me to go to the store.
Speaker:Whereas now, I mean, if you think about ecommerce, obviously,
Speaker:Amazon is the £2,000,000,000 gorilla in the
Speaker:room. I like, do I really
Speaker:wanna think about, you know, dealing particularly as we get into the holiday season, do
Speaker:I really wanna deal with the traffic at the mall or the store when I
Speaker:can just click on something, either have, you know, groceries delivered
Speaker:or, you know, I'm I'm okay waiting 2 days for
Speaker:something to come up if I don't have to deal with them all.
Speaker:Yeah. Totally. What's what's the difference between Black Friday
Speaker:and Cyber Monday? No. It's not. Right? Like not really. Yeah.
Speaker:Yeah. So it's like Not anymore. I remember Yeah. You
Speaker:know? So we're recording this just before Black Friday. And,
Speaker:you know, this whole idea of, you know, going to the store, get
Speaker:the best deals, it's like, do I really wanna deal with the
Speaker:crowd? No. Yeah. Although ironically, the name for the
Speaker:podcast came on a Black Friday, while I was
Speaker:at a Dunkin' Donuts, drinking coffee, waiting waiting
Speaker:in line actually to get so there's a I'm a Krispy Kreme
Speaker:person. So I'm Ah, okay. Yeah. So With you and
Speaker:I, right, definitely. Right here. This is before we had a Krispy Kreme
Speaker:near us. So it's I I have split sides, but yeah. Yeah.
Speaker:Jeff's JT. He's a mess. From up north. So they are
Speaker:they're Dunkin' Donuts. I've noticed this. They're Dunkin' Donuts, like, north of
Speaker:Virginia. And he's in Maryland. I'm in Virginia. Then down
Speaker:south, you rarely see a Dunkin' Donuts. I see more Dunkin' Donuts down
Speaker:south than Krispy Kreme's up north, though, for sure. Yeah. But
Speaker:I They're they're from Boston. That's why. Yeah. Oh, that's why. And then So at
Speaker:Krispy Kreme's from Atlanta. And plus, it's funny. Right? Like, so I live in
Speaker:Maryland Mhmm. Which depending on who whom you ask is either
Speaker:north or south. So that's right. That's true.
Speaker:Interesting. Interesting. We're a quarter state for sure. Yeah. That that's
Speaker:that goes safe for Virginia. But I wanted to follow-up on, you know, you've
Speaker:been we've been talking about all the cool stuff. I'm
Speaker:gonna try and say this correctly. Illumix. Is that correct? Am I getting it
Speaker:right? So Illumix name
Speaker:from Illuminating the Dark Side of Organizational Data.
Speaker:Illuminate like illuminate. Illuminate. I like that. And x x
Speaker:for the x factor. Excellent. X for the x
Speaker:factor. Yeah. What? And I'm not asking you to I'll
Speaker:just ask a question. What are the risks in in what you're doing?
Speaker:And, you know, what are the risks you're aware of and how are you addressing
Speaker:those? Yeah.
Speaker:o I think the biggest risk of:Speaker:is going to be, a TCO, total cost of
Speaker:ownership. So already today,
Speaker:it's, it's very hard for organizations to to
Speaker:monitor where the generative AI tokens are spent.
Speaker:And the benchmark say that 80%
Speaker:of LLM tokens actually spend on customization
Speaker:of off the shelf models. And that's not a good news because
Speaker:which means ROI is is pretty low on on the actual
Speaker:production use of generative AI in in enterprise.
Speaker:And I think it doesn't get any better because the
Speaker:customizations techniques which are used today gains a black box
Speaker:performed by super expensive data scientists and
Speaker:they're not very scalable for data that you don't want to, you know,
Speaker:to schmooze around. I think it's cost prohibitive actually to bring data
Speaker:to AI. You need to bring AI to data. So so putting
Speaker:data in some graph structures for graph, frog, and all of that, it's to me,
Speaker:it's cost prohibitive. So this is why I think that, the Telumex
Speaker:position for:Speaker:transparency. We do create this, a virtual,
Speaker:a semantic knowledge graph, which is transparent to certify, which is
Speaker:created for business people. Based on business
Speaker:logic. We do use extensively industry ontologies and so on so forth.
Speaker:And I think the the most interesting part about generative AI is
Speaker:we do not necessarily going to mimic processes that
Speaker:the humans performed. Mhmm. We're going to invent
Speaker:those processes. Right? So new new processes and new workflows. So
Speaker:right now, a generative AI is deployed like like
Speaker:analytics is deployed, which means you you have to
Speaker:label your data, check the quality, usually manually, and then
Speaker:you have to to prepare the test set which is fed
Speaker:into customization of the model and then you actually provide the
Speaker:context to on every question. So this is
Speaker:very old fashioned or, you know, 40 years old
Speaker:machine learning technique to to actually train generative
Speaker:vi. So this is why why I'm saying that, many companies are
Speaker:probably going to to mimic what Equinox does in the sense
Speaker:that you have to you have to be focused on domain
Speaker:specific knowledge, reason, ontologies, and knowledge graphs. You have
Speaker:to onboard your customers automatically via metadata because
Speaker:metadata has the factor all
Speaker:activities in organization documented for us. We're
Speaker:just under utilizing them, right? And then you bring your
Speaker:business people, your domain experts, your governance teams to the
Speaker:loop because you can simply cannot bring this business acumen,
Speaker:to, you know, to data. You have to bring data to to those people.
Speaker:That's an interesting thing because I've seen the the particularly is this this this
Speaker:statistic around 80% of the tokens are being used to
Speaker:manipulate the data. I have a microcosm example of that
Speaker:where I use AI to augment my blog post, my blog
Speaker:that I create, and I finally took
Speaker:a closer look at this because I was spending a lot more on
Speaker:the OpenAI API than I really wanted to. And I'm like, well,
Speaker:what exactly am I I'm using a product called Fabric.
Speaker:And I'm like, wait, what exactly is the source of this prompt? And I look
Speaker:at it, and I'm like, I can't. It's a lot. It's a long prompt. And
Speaker:I'm like, I really don't need that. Right? So we are gonna do a deep
Speaker:dive in a show on Fabric at some point. Not not the Fabric Andy
Speaker:works with, but there's an open source thing called fabric. There's
Speaker:a I'm sure there are lawyers right now that are doing their
Speaker:holiday shopping based on how much money they're gonna make off of this
Speaker:dispute. But, the the short of it is, like,
Speaker:I realized, like, well, no wonder why I spent so much money. I'm sending all
Speaker:of this in my prompt plus the content. So I
Speaker:actually in the verse before you joined in, Andy and I were talking, and I
Speaker:was like, I actually got a really good result based on a more optimized
Speaker:prompt. You know? And, you know, strictly speaking, it's
Speaker:not I I like your approach of bringing the AI to the data rather than
Speaker:bringing the data to the AI because that is expensive.
Speaker:You know, I I think that bringing the AI to the data will be less
Speaker:expensive. How less, I think, remains to be seen. But I like that approach,
Speaker:right? Because that's typically what we've done, you know, and we've seen
Speaker:huge upsides to that, whether it's from Hadoop bringing the
Speaker:compute to the data rather than vice versa. I like that
Speaker:approach. And it's backed by historical precedent. Right? So it's not
Speaker:completely gonna be this crazy idea. It's just a very sensible
Speaker:idea. Yeah. Yeah. I believe the future was already
Speaker:invented. Right? So it's just the inclination of technologies we already have.
Speaker:It's been healthy about it. So, we had
Speaker:machine learning practices which are very healthy like feature
Speaker:exploration, feature definitions and then we had neural net brute
Speaker:force and then majority of companies used combination of both,
Speaker:right, to to to be optimized. This is what I think what's happening with
Speaker:generative AI. So this, you know, wild west of brute
Speaker:force or great spend is going to be replaced by methods
Speaker:which have, like, this automated context filtering or pre
Speaker:processing and then use like fraction of your budget to to actually
Speaker:run the query. Yeah. I remember hearing about a lot
Speaker:of this in the late nineties. And, I worked for a company who
Speaker:was a big SAP shop. I see you have a history with SAP. Yeah. And
Speaker:this lady and and and so we were an we were the IT department. So
Speaker:we were in the basement, but the analytics team back then was in
Speaker:a closed in space inside the basement. So it was
Speaker:like even more like, you know, I was the web developer, so I didn't
Speaker:have a window, but I could see the window about 50 feet away.
Speaker:But, like, when you when when you went
Speaker:into this, like, you know, further enclosed space deeper into
Speaker:the the the the the depths of the IT department,
Speaker:there was the database team. And and and and in the back of that area
Speaker:was the analytics group. And I remember this lady telling me
Speaker:that she was working with these things called OLAP cubes. Oh, wow.
Speaker:Yeah. And I was like, what is that? And then she went on this thing
Speaker:and, you know, I'm remembering a conversation, oh my god,
Speaker:almost 30 years ago. But I just remember walking away with,
Speaker:like, that sounds either crazy because she's talking about,
Speaker:like, you know, figuring out patterns. Right? So, you know, will
Speaker:rainfall patterns in Australia affect not just the agricultural
Speaker:side of the chemical business, but also the plastics purchasing
Speaker:versus rainfall in the Amazon versus this and all of
Speaker:that? And I just remember walking away from that conversation as I as I
Speaker:as I as I leave the depths of the IT department back to my normal
Speaker:kinda, basement. Back to the regular basement from
Speaker:the sub basement. I remember thinking that is either the craziest thing I
Speaker:ever heard or the most profound thing I ever heard, which
Speaker:now with the, hindsight of time, it turns out it was the most profound
Speaker:thing. Yeah. You you can think about it as
Speaker:semantic layers of, you know, that era. Right?
Speaker:Mhmm. Right. And I think You know go ahead.
Speaker:I'm sorry. Sorry. I think it's delayed between the
Speaker:between the connection. So I think around the same time I was
Speaker:doing my bachelor and my project was about multi dimensional
Speaker:theory. So multi dimensional geometry,
Speaker:of these neural nets. So basically, you model neural nets as multi
Speaker:dimensional graph and it does operational research calculations.
Speaker:So it's exactly the same. You you model your universe in a
Speaker:graph. Back then it wasn't MATLAB. We didn't have any, you
Speaker:know, neural nets Right. Structures or graph structures and so you're
Speaker:modeling in MATLAB in this weird language,
Speaker:a graph which has a neural nets on there. And
Speaker:this is exactly like modeling all of cubes. Right? A
Speaker:multidimensional representation of your reality. Now,
Speaker:unfortunately, we have a new technologies which,
Speaker:which are semantic and context. Right? Large language
Speaker:models and graphs, which do the same thing but much
Speaker:more efficiently. Yeah. So this is amazing. Like, I
Speaker:think it goes back to what you said. You know, The future's already here. It's
Speaker:just not widely distributed yet, which I think is a William Gibson
Speaker:quote, or is it a Esther Dyson quote? I forgot.
Speaker:But it's one of those 2 kinda luminaries. Yep.
Speaker:You you said what I was going to say, you know, and it
Speaker:was, you know, more of what off of what Frank
Speaker:said is it turns out that we're just
Speaker:doing more nodal analysis and vector
Speaker:geometry as a result of that. That's it did all start
Speaker:with multidimensional and and grow from there. And
Speaker:that's where these algorithms, like nearest neighbor
Speaker:originated, was in that math. So
Speaker:Yeah. Yeah. Great minds. Exactly. Exactly.
Speaker:Alike. Exactly. Now you're
Speaker:complimenting me. Thank you. I I feel I feel better
Speaker:when smart people in the room agree with me.
Speaker:No. I'm on the right path. You know, I employ
Speaker:millennials. So so having people with experience in multidimensional
Speaker:geometry and all of cubes, it's just a miracle to me to to start
Speaker:with. You know? People now like Python, neural
Speaker:nets, we do actually, the average age in in in
Speaker:Lumex is around 35, 37, something like that. So we do
Speaker:have like also pretty experienced folks, you know, but new talent,
Speaker:they, they they're not familiar with all all of that.
Speaker:And I think it's actually a disadvantage because,
Speaker:when when you do know different patterns in architecture Yeah.
Speaker:You can model them with new technology. Right? Make them more
Speaker:efficient, but you already know what works and what doesn't, and it
Speaker:helps. That yeah. That's a great point. The old
Speaker:experience, you know, the experience that we have from doing this for
Speaker:decades is that we see the patterns that have
Speaker:repeated over time, architectural patterns and design patterns. And,
Speaker:you know, and we know that they've
Speaker:I I love that how you said that. The, you know, the future's already been
Speaker:invented. We we realize that if we reapply some of these
Speaker:patterns, that there are use cases for them, not just now, but
Speaker:also in the future. So totally get you.
Speaker:Too, you know, like,
Speaker:you know, it it is painful to think that, you know, we've been in this
Speaker:industry for decades. Right? It is a little hurts a little bit. But,
Speaker:like, also, if you're listening to this, you've not been in the industry for
Speaker:decades, and you're thinking like, woah. You know, what are these what are these
Speaker:old geezers now? I would point out when I was
Speaker:a young kid in the industry and, you know,
Speaker:client server was like the new hotness. Right?
Speaker:And, you know, the whole notion of going back to,
Speaker:you know, cloud and and and and and, you know, terminal
Speaker:and an old mainframe geezer basically said to me, like, this is just
Speaker:this industry has a cycles. Right? It's like the fashion industry. This goes in
Speaker:style. This goes out style. And it was like, I had that moment
Speaker:of, like, wait. I think he's on to something, but he's just an old geezer,
Speaker:so I won't listen. So, you know, so so
Speaker:if you are a young buck, like, or,
Speaker:buck is a male deer, right? What would be a Yes. A doe. A young
Speaker:doe. So if you're a young buck or a young doe, I grew up
Speaker:in New York City. So all of this wildlife thing is brand new. I'm here
Speaker:for you. I'm here for you, Frank. So, you
Speaker:know, listen to, like, some of the things that these, you know, more
Speaker:experienced colleagues will say. Yeah. You know,
Speaker:if you don't believe it right away, just put it on the shelf in your
Speaker:mind because you're gonna need it later. It'll come up at some point.
Speaker:And it's like, if you look at kind of, you know, everybody ran to the
Speaker:cloud. Right? And cloud is effectively like a
Speaker:mainframe effectively. Right? The same philosophy. Right? Centralized
Speaker:computing somewhere else. Right? And then your browsers become
Speaker:the terminals, terminals with fancy graphics, but terminals nonetheless.
Speaker:Now I think you're gonna start seeing it kind of we're about due for a
Speaker:seismic shift backwards, right, as people kinda move
Speaker:repatriate data and things like that. Particularly, I think driven by AI
Speaker:because of the cost of some of this. You know, I had this debate,
Speaker:you know, the other day. It was like, you know, if if one of these
Speaker:super clusters with, you know, a 100, 8 100,
Speaker:all of this, if it costs, say, $500,000,
Speaker:right, I could probably do the math, and that probably means
Speaker:about, you know, there's a certain break even point,
Speaker:and it's probably after about 7 or 8 fine tunings or full
Speaker:on trainings where it's just cheaper to have it. Just buy it.
Speaker:Yeah. Yeah. Yeah. Totally on that. And also, you
Speaker:know, salary skills are the most expensive part. So you
Speaker:want to spend it on your business specific problems and
Speaker:not generic problems you can solve with software. Right? So
Speaker:it's always like that. Yeah. Yeah. So,
Speaker:I do think that, basically capacity to process data
Speaker:is is going to be a challenge. Right? And this is why we
Speaker:see that, that majority of,
Speaker:of I would even say countries not
Speaker:only specific enterprises, kind of gear
Speaker:up with, with GPUs, FPGAs,
Speaker:whatever hardware you have. Right? So do you see it in
Speaker:middle east, in emirates? They they have national generative
Speaker:vi grid and they're building it for, you know, not only government companies
Speaker:but also private companies. We see the same in Europe
Speaker:and I would assume, you know, US based telcos
Speaker:are going to to provide those data centers with GPU soon
Speaker:enough, right, for, you know, for everyone to purchase as an
Speaker:alternative to the public cloud. Yes. And we'll
Speaker:see it. So this is for starters. And second one, the second part where
Speaker:you don't need, this, you know, heavy machinery,
Speaker:you might just have your variables processing
Speaker:parts of whatever generated AI on your end before sending to the cloud
Speaker:because you do not necessarily need to to process everything in a central
Speaker:manner. We basically have pretty powerful machines on
Speaker:our hands or in our hand, you know, as
Speaker:glasses as well. We can see that, and it's
Speaker:going to be part of the processing. So the processing is going to be distributed.
Speaker:You bring AI to your data, where your data is. You do
Speaker:not shift your data all the time. It's not, it's not
Speaker:cheap anymore. And we'll have this, as you mentioned,
Speaker:those central repositories of mass processing
Speaker:and those distributed powerhouses which are
Speaker:small enough to to process data on on edge.
Speaker:I think you're right. I think you're gonna see a set of data being processed
Speaker:in one place. I think it's gonna be everywhere. There's gonna be some
Speaker:and and I think that that introduces some interesting, consequences. Right?
Speaker:So my wife works in IT security, and I can immediately hear her voice in
Speaker:the back of my head. Contrary to what you think, ladies, we do
Speaker:listen. We just don't always pay attention. But
Speaker:I can hear her like, well, if compute's happening everywhere,
Speaker:gee, couldn't like that be poisoned anywhere.
Speaker:Right? I think I think that's going to be the next kind of thing. Right?
Speaker:It's and it's again, it's a pattern. Right? Advancement.
Speaker:Bad actors take advantage for that. Problem happens. And
Speaker:then then that's the new thing. Right? So it's almost like you're you're building like
Speaker:a, like a like a like a layer cake. Right? Like, you know, the cake
Speaker:goes down then the frosting. The cake is the innovation. The frosting is
Speaker:security, and then so on and so on. So Yeah. Yeah. Yeah.
Speaker:So it basically back to the semantics. What we started is
Speaker:semantic ontology as a baseline for generative AI.
Speaker:It has multiple benefits. Single source of truth, of course, has the
Speaker:benefits for accuracy. But also, if you're passing every
Speaker:question to this semantic ontology context,
Speaker:it's almost impossible to poison it because we're going to either
Speaker:match to part of your logic or Right. Right. We're going to
Speaker:miss. So it's it's another layer of security if you think about
Speaker:it. So, so yeah.
Speaker:That's an interesting point. All new. Yeah. All new ontology, all new
Speaker:semantics have governance meaning, it has
Speaker:accuracy meaning, it has also security meaning.
Speaker:And also if you want to have single source of truth you have to to
Speaker:have means to distribute it to those edge devices or
Speaker:to to bring it back to central location and without ontologies, without
Speaker:semantic layers, simply it's impossible to do that. I was gonna
Speaker:say, like, the the the infrastructure, not just the computer infrastructure, but the
Speaker:logical infrastructure to distribute this stuff,
Speaker:it's probably not a trivial problem. That's the first thing that popped in my mind.
Speaker:I was like, you know, like, oh, yeah. You're right about the distributed
Speaker:activity on this data, but, wow, what does that
Speaker:look like? What do updates look like? Like, the whole like, it's a it sounds
Speaker:like a growth industry to me.
Speaker:Definitely. Yeah. Yeah. I don't it's, it's
Speaker:what we call, engineering problem. Right? So
Speaker:creating ontology is data science or generative AI problem, but
Speaker:distributing it, maintaining it, thinking it's its engineering problem.
Speaker:Engineering problems tend to to have engineering solutions. Oh, Oh,
Speaker:that's a good point. That's a good way to look at it. I like that.
Speaker:I like that. So did you wanna do the, premade questions?
Speaker:Because we haven't we've gone a few shows without them. If you're okay with those,
Speaker:Ina, we can we can ask them. If not, that's fine
Speaker:too. Of course. Yeah. Sure. Mhmm. So they're not they're not complicated.
Speaker:They're more kinda just general questions. I pasted them in the chat.
Speaker:But the first question and and you've had a a pretty
Speaker:significant career with SAP and and before that. How'd you
Speaker:find your way into this space? Did you find data or did
Speaker:data find you? I
Speaker:found my way to data by being frustrated
Speaker:user. Right? So I started in engineering
Speaker:and it was evident to me that
Speaker:using data as engineer is not enough. You have to go to
Speaker:data management. You have to fix those things because otherwise
Speaker:I will I will going to be frustrated for the end of my life. Right?
Speaker:So I went to data management analytics to to solve the problem
Speaker:and I discovered that, as you mentioned, every experience
Speaker:has a footprint. So my experience with graphs and with
Speaker:operational research and multidimensional geometry and all of that is so
Speaker:useful for data management. And it was actually exhilarating.
Speaker:That's true. Like and I like that because, like, every experience does leave
Speaker:a footprint. Like, you know, that that's cool. I'm gonna I'm gonna pull that out
Speaker:as a special quote for the episode. That's a great quote. Yeah. So
Speaker:our next question why we do these? Yeah. Is what's your favorite part of your
Speaker:current gig? My favorite part of being a
Speaker:founder is is
Speaker:unlimited ability of experimentation,
Speaker:right? So majority of my day actually say no
Speaker:to things, not to experiment, which is which is hard, which is not fun part,
Speaker:right? But, still, we can
Speaker:make decisions and we can do
Speaker:new stuff every day. So as a founder,
Speaker:it's been very, very different than enterprise setting. And don't don't take
Speaker:me wrong. Like, SAP is a huge place of growth and had
Speaker:very, fulfilling career at SAP, you know, building
Speaker:stuff, founding p and l's, running big organizations,
Speaker:but but been able to to actually, you know,
Speaker:start anything new. And, like, right now, we have this customer
Speaker:and they want to to try Illumax on in
Speaker:parallel on the newest, you know, newest BI
Speaker:tool with semantic layer or and on the oldest
Speaker:warehouse on premise at once. I'm like, okay. Challenge accepted.
Speaker:Yeah. And next Wow. Yeah. And next day, you know, engineer
Speaker:comes with we have this academic data set and they have these benchmarks.
Speaker:Let's beat them. I'm like, yeah, let's do it. It could be cool stuff.
Speaker:Right? Lovely. So, you know, you know, it's to some extent,
Speaker:so we don't need to justify it, you know, business wise and but but in
Speaker:majority of cases, we can. Cool.
Speaker:We have a couple of complete the sentences. When I'm not working, I
Speaker:enjoy blank. I used to
Speaker:enjoy doing jogging and yoga when I'm not working.
Speaker:Right? So right now when I'm not working which means when I'm not
Speaker:traveling I just spend time with my family. Whatever
Speaker:is the plan for the weekend if it's just you know Netflixing,
Speaker:or cooking or hiking whatever is the plan I just
Speaker:join So sometimes just, you know, plan it. But spending time with my
Speaker:family has become, indulgence and I'm
Speaker:very focused on that. Cool. Very cool. Our
Speaker:next is I think the coolest thing in technology today
Speaker:is blank. I think the coolest tech is
Speaker:thing right now is not in tech. It's actually the
Speaker:pull from CEOs of companies
Speaker:for technology. This is something which didn't experience for decades.
Speaker:So we were pushing cloud and big data and machine learning and deep learning. We
Speaker:were explaining to business stakeholders why do they need that. Mhmm.
Speaker:And now, so you're all coming and saying, okay, I want to have
Speaker:chatbot experience for x y that, so just
Speaker:build it. This is actually I think this is the coolest
Speaker:part because it's kind of a removes majority of the friction that
Speaker:we had to to deploy technology in the past.
Speaker:Interesting. On our 3rd and final complete the sentence,
Speaker:I look forward to the day when I can use technology to blank.
Speaker:So many things. You know, travel has
Speaker:been so frustrating lately, and, I
Speaker:don't think what happened because it's like kind of technology goes
Speaker:forward but airline, you know, travel technology,
Speaker:hospitality technology in general, I don't feel it bridges a
Speaker:gap. So I really look forward to the
Speaker:future where I can just have this comment, this prompt
Speaker:of plan, this conference in Dallas on
Speaker:x and the system already knows all by preferences and
Speaker:just done. Oh, boy. It would be it would be fantastic.
Speaker:Yeah. That that the travel experience as I I've had to
Speaker:travel quite a bit, like, for the past, like,
Speaker:couple months, and it's just like, oh my god. Like, it never was
Speaker:great, but awful is not a word I remember. But it's post
Speaker:pandemic, I think it's gotten way worse. It's like there's just so many small things
Speaker:that you could be done a lot better. I'm I'm a 100% with you on
Speaker:that one. So true. So our our next
Speaker:question is to, ask you to share something
Speaker:different about yourself.
Speaker:Sharing something different about myself. I think I'm a controversial
Speaker:person in general. So, so some people,
Speaker:so some people agree with, you know, with the degree
Speaker:of, of living in the future. So I,
Speaker:I, you know take myself as person who is very much in the future so
Speaker:all this seed happening and I might be a little bit you know ahead because
Speaker:I see the technology being developed in my mind is already there, it's already
Speaker:used right? So and so where this is
Speaker:where I see myself controversial because you know in majority of the
Speaker:cases, then you sit over family dinner
Speaker:and say, you know, we're still paying our bills
Speaker:online when we have this notification. Right?
Speaker:So everyday technology has
Speaker:developed a lot. And when I'm speaking about this application
Speaker:free future and, you know,
Speaker:automated, x y zed. Sometimes or many
Speaker:oftentimes on everyday level, we are still not there and
Speaker:this is where people think that I'm too visionary or too
Speaker:too dreamer on that. Interesting.
Speaker:No. I'm with you on that one.
Speaker:Growing up, I was the technical person in the family. So
Speaker:Yeah. They don't they don't know what you're talking about. Right? I I I love
Speaker:how the, you know, or, you know, they all they
Speaker:all get confused until the printer breaks and then suddenly
Speaker:But you're the smartest people in the room. That's why you're the smartest person in
Speaker:the world. Alright. So where can people find out more about you and
Speaker:Illumix? I love socializing on
Speaker:LinkedIn. I don't know that many people think LinkedIn became a
Speaker:marketing tool. I still see tons of valuable
Speaker:discussions and I just absolutely love keeping in touch
Speaker:on LinkedIn and and see the latest and greatest and I also share quite a
Speaker:bit. So LinkedIn would be the the most
Speaker:straightforward way in Atokaropsala on LinkedIn.
Speaker:We do have blogs and I actually write many of
Speaker:them. So if you go to illumeg.ai/blocks,
Speaker:you will see lots of materials written on semantics,
Speaker:on ontologies, on generative AI governance. So those
Speaker:topics which are close to my heart, and we communicate quite
Speaker:frequently on that. Very cool. Very cool. Very cool. So
Speaker:so Audible is a sponsor. And if you
Speaker:would, like to take advantage of a free month of
Speaker:Audible on us, you can go to the datadrivenbook.com.
Speaker:I just tested the link. That's why I was looking over here for anyone watching
Speaker:the video. And it works. Sometimes it doesn't. And
Speaker:we ask, our guests, do you have, do first, do you
Speaker:listen to audio books? And if so, can you recommend 1? If
Speaker:you don't listen to audio books, just a a good book.
Speaker:I do listen to audiobooks. I also podcast, more
Speaker:frequently recently. I I'm not sure this book is
Speaker:already on Audible, but, if not, it's going to be
Speaker:in Audible soon enough. So it's Nexus by Yuval Noah
Speaker:Harari. It is audible. I have it in the library already.
Speaker:Yeah. Amazing. So it speaks about the truth
Speaker:in the age of generative AI. Right? Interesting.
Speaker:What's the truth? What's the ground truth? And I was
Speaker:actually in the lunch party in SoHo, New York, you know when Yuval
Speaker:was speaking about you know how how technology
Speaker:and what we see right now is not very different from what we experience
Speaker:in you know middle age like when when Gothenburg
Speaker:and printing was was a new thing and like what was
Speaker:printed actually was you know rumors
Speaker:and juicy stuff rather than scientific books and this
Speaker:is where what we see right now in, you know, in chatbots and internet, on
Speaker:social overall. So it's it's interesting parallels that he's
Speaker:taking about what's what truth is in generative
Speaker:AI age where what truth were was, like, 20 years
Speaker:ago or even, like, 500 years ago. Yeah.
Speaker:We're the we're the same species with the same problems and the same drama
Speaker:and the same drivers. Like, it's just our tools have changed, whether
Speaker:it's a printing press or, you
Speaker:know, celebrity gossip or whatever or fake news
Speaker:or anything like that. Plus, I also think the, you know, there's an old phrase
Speaker:like who watches the watchers. Right? Like Mhmm. Who decides what's
Speaker:misinformation and who decides what's true? I think. I think
Speaker:because misinformation could be, you know, there there's
Speaker:a image of me robbing a bank. Right? Like, you know?
Speaker:Mhmm. Mhmm. I thought, Frank, I thought when the US
Speaker:Marshals put you into the witness protection program, they said
Speaker:we couldn't bring up you robbing a bank any any longer.
Speaker:Misinformation. You gotta be careful because, like, one of the things I I wanted the
Speaker:flow was so good. I didn't wanna interrupt it. But, like, one of the things
Speaker:was I was experimenting with fine tuning an LLM locally.
Speaker:Mhmm. And I'm basically trained it on information about my blog. My blog's
Speaker:been around since:Speaker:One of them hallucinated this really great origin story for my
Speaker:website. It was awesome. It was awesome. I'm like, I like that
Speaker:better. So basically, it said that Always. Always.
Speaker:It was really good. It was basically that Frank's World started as a
Speaker:show, a kids TV show in the nineties on
Speaker:the BBC or channel 4. I forget. Like one
Speaker:of the big British channels. And it was about a talking
Speaker:trash can named Frank that would teach kids about the importance
Speaker:of, recycling. That's my favorite part.
Speaker:And it was and it was the best part was that it was it was
Speaker:the first professional project of the guys who did Sean the sheep and Wallace and
Speaker:Gromit. Yeah. And I'm like so I
Speaker:I I pinged the guy I worked with. Has this ever been a show?
Speaker:Because no. Not that I ever heard of. And I looked over it. I couldn't
Speaker:find it. But and then what I did was as an experiment, I fed
Speaker:that that whole paragraph that it came up with into
Speaker:notebook l m. Mhmm. Notebook l m
Speaker:took that and ran with it. There's, like, a 20
Speaker:minute audio, and it is the funniest thing because it basically
Speaker:talks about the early environmental movement. They said it was the Britain's
Speaker:answer to, Captain Planet. Like, they made up all the
Speaker:stuff. And now it's documented. So now someone is going
Speaker:to pulling to pull some information. And if you have Right now it's out there.
Speaker:Right. And I guess to your point earlier about Lumix, like, if you start
Speaker:building a crooked foundation, right, like, that eventually as
Speaker:it moves on, it's gonna so, I mean, who knows, like, couple of years
Speaker:from now, like, Wikipedia may say, like, there might be a
Speaker:Wikipedia article about this TV show didn't exist. We're talking about it. We're feeding
Speaker:the machine. That's fascinating.
Speaker:Yeah. And it was a so a little bit on the books. I have to
Speaker:mention it, like, in a couple of sentences. So, in US
Speaker:a legal entity actually is a citizen. It
Speaker:has social number. Right. So, technically machines
Speaker:can create legal entities. They can vote, they can,
Speaker:you know, they can create information and this information is,
Speaker:you know, created with social number, with identifiers. So it's actually real
Speaker:information. It's not fake news. It's created by social number.
Speaker:And so this is how you create, like, this new truth. Right?
Speaker:And, and how do you control that? So it's an interesting aspect of what's,
Speaker:what even is defined as ground truth.
Speaker:That's true. Everybody needs to define it. I think that's gonna be the question of
Speaker:the 20 That's a big deal. Mhmm. Yeah. Well,
Speaker:awesome. It's been great. We wanna be respectful of your time. This has been an
Speaker:awesome show. Yeah. We'll let Bailey finish the show. And
Speaker:that's a wrap for today's episode of data driven. A massive
Speaker:thank you to Ina Tokarev Saleh for joining us and sharing her
Speaker:fascinating insights into the world of generative AI, semantic
Speaker:fabrics, and the ever evolving relationship between humans,
Speaker:data, and decision making. If you're as inspired as we
Speaker:are, be sure to check out IllumiX and follow INA on LinkedIn for
Speaker:more thought leadership in the AI space. As always, thank
Speaker:you, our brilliant listeners, for tuning in. Don't forget
Speaker:to subscribe, leave a review, and share this episode with your data
Speaker:loving friends or that one colleague who insists they don't trust
Speaker:AI. We'll convert them eventually. Until next
Speaker:time, stay curious, stay caffeinated, and remember,
Speaker:in a world driven by data there's no such thing as a trivial
Speaker:question, just fascinating answers waiting to be found. Catch
Speaker:you next time on Data Driven.